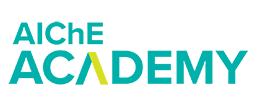
The Definitive Resource for Engineering Education
At every stage of your career, AIChE Academy is the definitive resource engineers use to sharpen their professional skills. We offer up-to-date courses and webinars in chemical engineering, process and hydrogen safety, bioengineering, sustainability, professional development, and many more.
Live Courses & Webinars
View CalendareLearning
View AllOn Demand Webinars
View AllExplore More Areas of Advancement
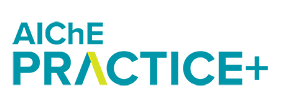
AIChE Practice+ provides learners with opportunities to work on real-world challenges through industry internships and competitions.
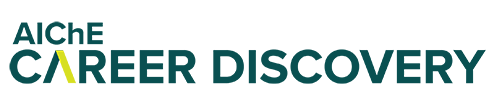
With AIChE Career Discovery®, we'll help you to identify aptitudes and skills you’ll need in order to achieve your full potential at various career stages.
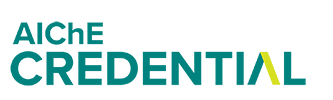
AIChE Credential validates your proficiency with potential employers in areas such as process intensification, safety, sustainability and others.