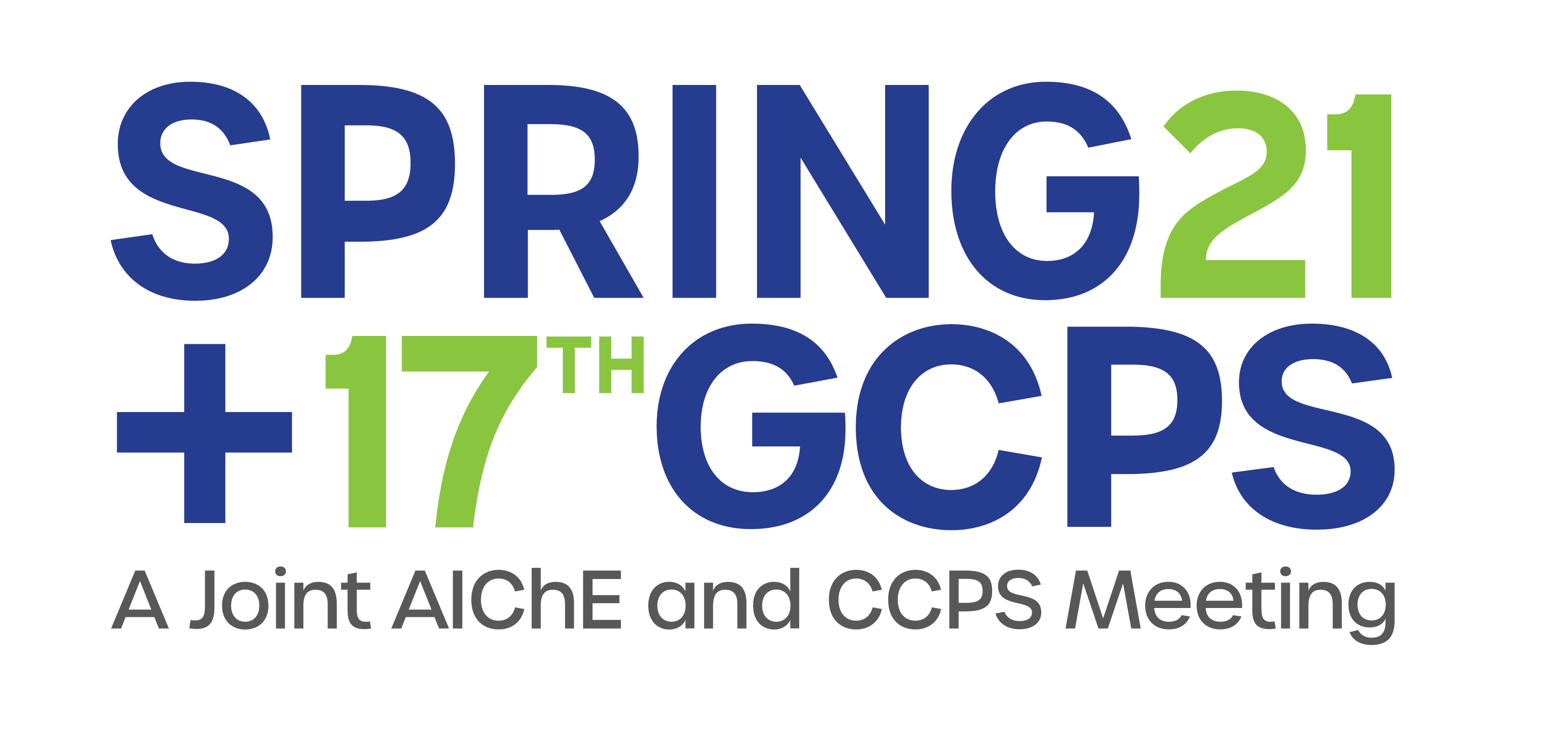
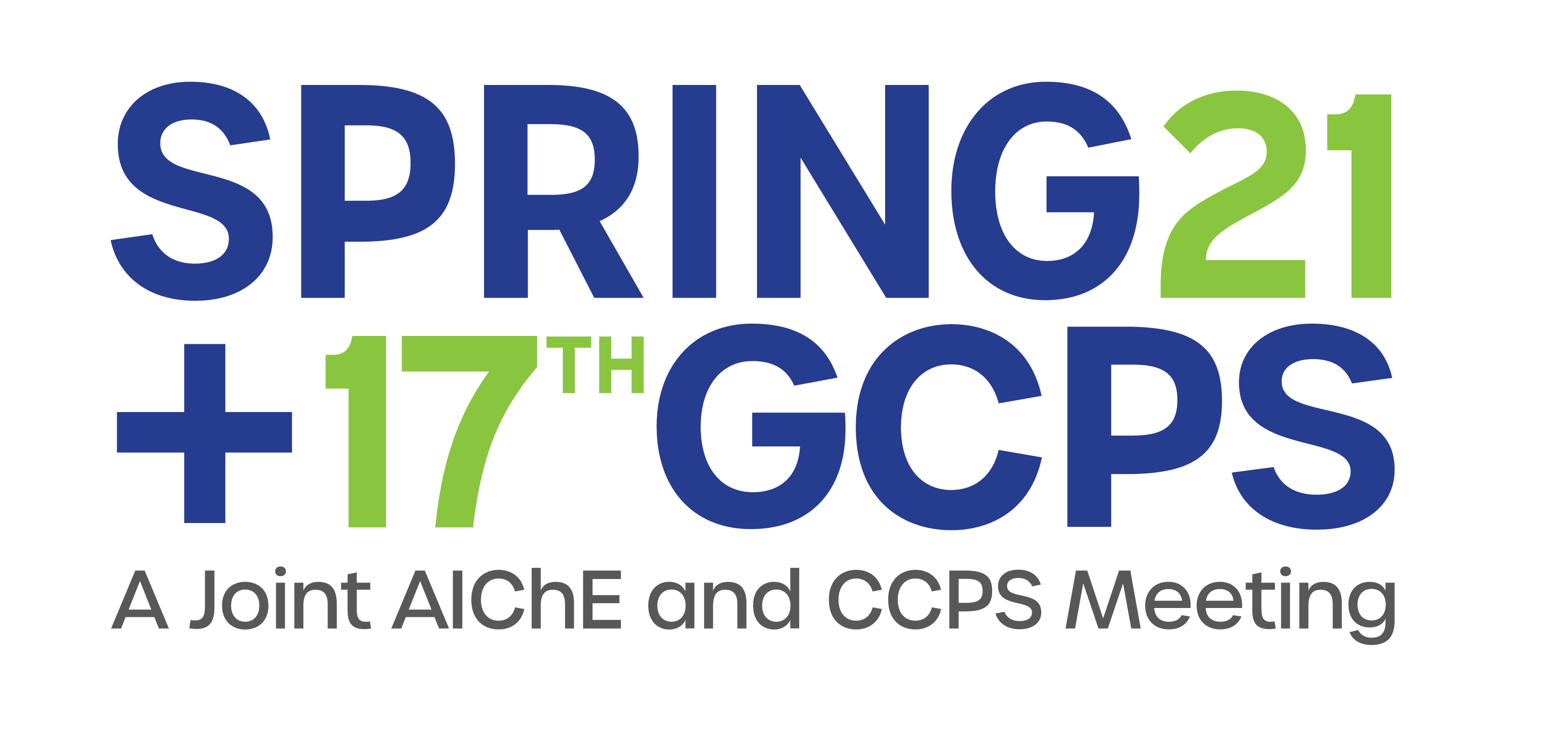
Causation analysis of pipeline failure using past incident data has been in practice for direct causes, such as corrosion, excavation damage, and equipment failure for more than three decades. However, the key to learning from past incidents is to identify the underlying causes of the incidents, rather than direct causes. Existing approaches for utilizing past failure data are not able to fully capture the dependencies of both direct and underlying causes and their contribution to the pipeline failures. There is a need to understand the synergy among direct and underlying causes and their collective ability to affect the integrity of the pipeline. Although a wealth of empirical knowledge has been accumulated from public incident databases and incident investigation reports, learning is still limited due to the high expense of manual content analysis and lack of appropriate techniques to gain insights from past incidents. This research is aiming using artificial intelligence for understanding causal relations for direct as well as underlying causes behind the incidents. Natural language processing (NLP) techniques, both unsupervised and semi-supervised learning algorithms, are being employed to enable automated content analysis and extract causal relations articulated in the incident reports. The techniques are capable of identifying dependencies among the direct causes, underlying causes and other contributing factors behind the incidents. An artificial neural network (ANN) based causal reasoning model is also being developed utilizing all relevant factors for pipeline failure reported in the pipeline incident database and elsewhere, and causal relationships identified by the NLP technique. The advantage of using ANN is that it does not oversimplify the causal relationships leading to identification of both linear and non-linear components of causality and its non-imposition of restriction on the input variables (such as their distribution). The combination of NLP and ANN models will improve the ability to make full use of vast data resources of pipeline incidents.
Preview Presentation
Presenter(s)
Once the content has been viewed and you have attested to it, you will be able to download and print a certificate for PDH credits.
If you have already viewed this content,
please click here
to login.