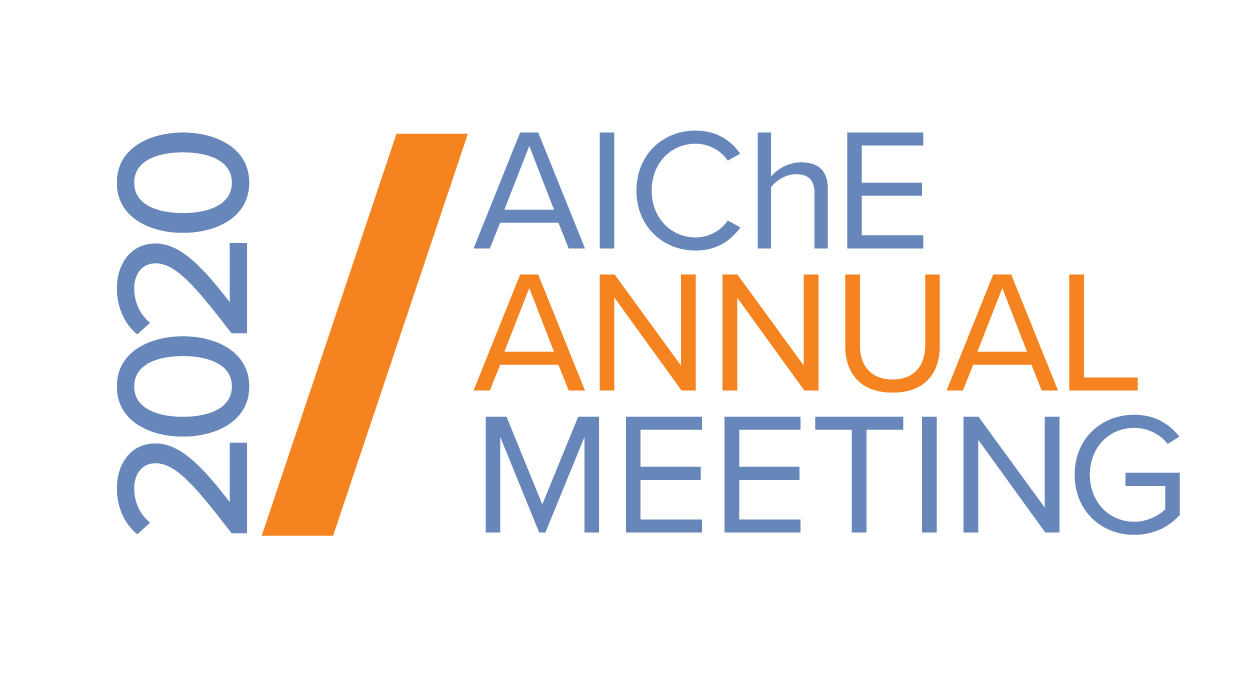
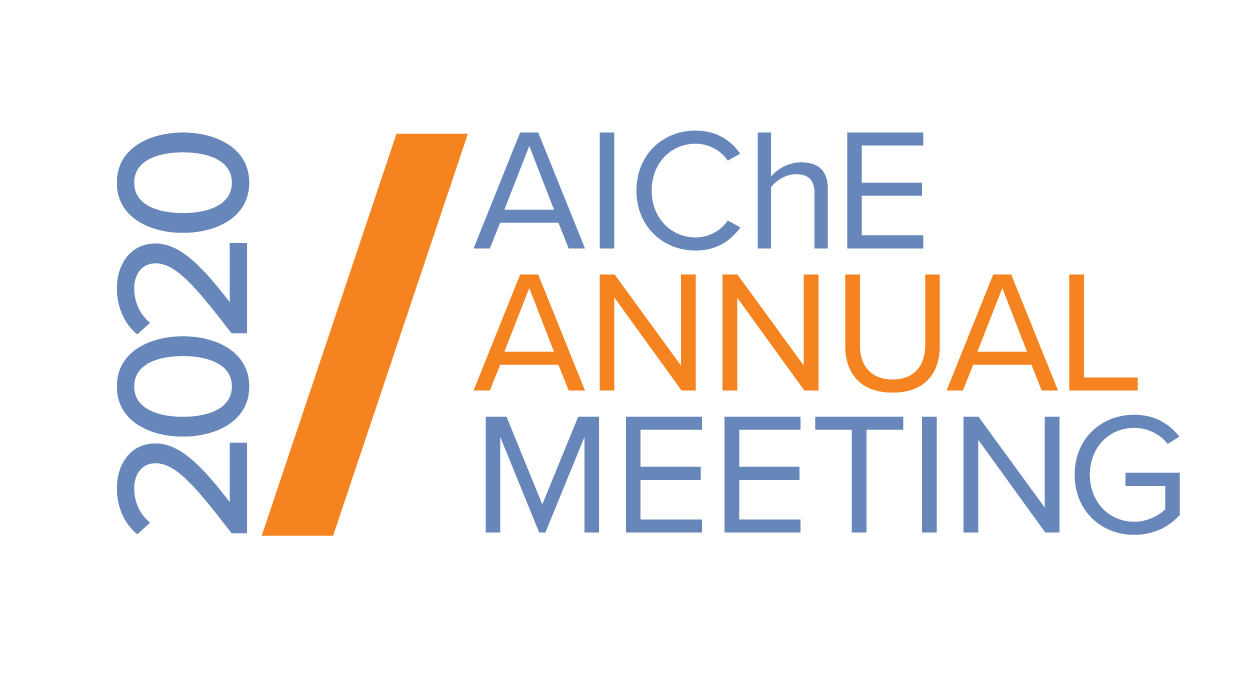
To address this problem, recently, the development of the factor analysis system by using process data from sensors installed in the production line has been promoted[1]. In the present system, features of sensor data are statistically analyzed and the degrees of influence of each feature to the product quality are scored. Therefore, the sensors which mostly affect product quality can be extracted. However, on the present system, the operators need to estimate the factors of defects and determine the countermeasure based on the extracted sensors because the present system does not automatically select factors of defects and countermeasures. To solve this issue, we have proposed the algorithm that discriminates factors of defects and determine the appropriate countermeasure by using process data and past countermeasures record.
In the proposed algorithm, firstly, countermeasures implemented in production line are linked with factors of defects extracted by Fault Tree Analysis of manufacturing process in advance. Secondly, various sensors related to the factors are selected. Thirdly, for each factor, the regression model of the factor occurrence is constructed by Gaussian Process Regressor. Here, the normal state data where no defects are generated are used as training data. Finally, the anomaly score of the factors are calculated during manufacturing, then the factor with the highest anomaly score and appropriate countermeasure can be determined.
We applied the proposed algorithm to the highly-functional film production line. As a result of verifying the algorithm by using actual process data, it was confirmed that the accuracy of the predicted countermeasure was higher than 90%. By automating the determination of countermeasure by using the algorithm, downtime for countermeasure is reduced and productivity is improved.
Reference
[1]Haifeng Chen, Mizoguchi Takehiko, Yan Tan, Kai Zhang, Geoff Jiang, proceedings of the 2015 45th Annual IEEE/IFIP International Conference on Dependable Systems and Networks, 529â536, 2015