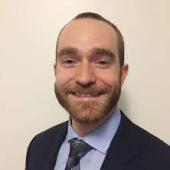
Understanding material behavior is critical element of identifying viable chemical & process routes, catalysts, and operating conditions necessary to facilitate and control the chemical & physical transformations employed to safely, consistently, and sustainably convert raw materials into desired products. We rely on measured process data, analytical results, and model output results to inform process & technology development and enable process system operation. The process insights and understandings obtained from these sources are essential to informed tactical operating decisions, targeted innovation, and business & technology strategy. Correct interpretation of this information has the potential to open the door to improved understating of the desired physical & material system; however, inferring behavior from erroneous data, incorrect results, and physically impossible model output offers consequences that range from wasted time to catastrophic disaster. Assessing the quality of process data, analytical, and simulation results and recognizing the differences between good & accurate information providing previously unrealized insight(s) and output from damaged instruments, corrupted signals, contaminated samples, and poor or missing material property system interaction parameters presents a continuing challenge for the process development and operating teams. Presentations in this session will discuss the collection, interpretation, use, and impacts of process data, analysis, and process modeling results to inform process & technology development and process operation in the laboratory, at pilot scale, and in production plants.
Session Chairs:
- Onkar Manjrekar, AbbVie
- Liz Moore, RAPID
- Stephen Tieri, DuPont
Tentative Schedule:
Time (EDT) | Presentation | speaker |
---|---|---|
1:35 PM | Pilot Plant Scale Execution and DOE Study of a multi-step Flow Process for a Pharmaceutical Intermediate | Travis Armiger, Merck |
2:05 PM | Integrating Multiscale Modeling and Optimization for Sustainable Process Development | Xin (Frank) Zhu, Honeywell |
2:35 PM | To AI or Not to AI, is Part of the Loaded Question |
Chris Clayton, Beyond Limits |
Abstracts:
Pilot Plant Scale Execution and DOE Study of a multi-step Flow Process for a Pharmaceutical Intermediate
Travis Armiger, Merck; Robert Franklin, Merck & Co., Inc
Flow chemistry is an attractive option to develop processes that may be difficult or impossible to execute in traditional batch mode. It is important to consider the challenges and opportunities associated with flow chemistry as it is scaled up from the bench to the commercial scale. This presentation will highlight the production of a pharmaceutical intermediate, using a plug flow reactor system, which was successfully demonstrated at the 20 kg scale in the Merck Small Scale Organics Pilot Plant in Rahway, New Jersey. The process involved an initial batch reaction, followed by a multi-step flow process and subsequent isolation. The set-up required the use of 5 vessels, 3 feed streams, 2 static mixers, and 5 heat exchangers with target stream temperatures below -20°C. Throughout the system temperature, pressure, and flow rates were monitored in real time, throughout the process to ensure that steady state was maintained. Additionally, an inline FTIR was installed for data collection at the end of the flow reactions. The system was designed to accommodate between-batch flushes, start-up and shutdown operations and upset scenarios. Five batches were successfully executed, with the 5th batch including a large-scale Design of Experiments (DOE) study. Due to the nature of flow processing, it is possible to rapidly change reaction conditions (temperature, flow rates) to allow for this DOE-type experiment to be performed at the pilot plant scale. This DOE enabled experimental data to be collected at scale at a variety of conditions which was then leveraged to determine the differences between the lab- and pilot-scale performance. Ultimately the knowledge gained from these data rich experiments allowed the team to confirm the feasibility of utilizing a bench scale plug flow reactor to set the proven acceptable ranges for process characterization. This presentation will highlight the operational lessons learned from scaling up a complex flow process, the value of real time data acquisition, and the insights of DOE experiments at scale.
Integrating Multiscale Modeling and Optimization for Sustainable Process Development
Xin (Frank) Zhu, Honeywell; Lijun Xu, Honeywell
The new approach for process development proposed in this article is based on integrated molecular modeling, process integration and mathematical optimization. Molecular modeling is about achieving the best molecular transformation to maximize desired products and minimize by-products, which is accomplished via reaction chemistry optimization and catalyst development. Process integration is about selecting fit-for-purpose technologies for reaction, separation, and heat transfer systems, while mathematical optimization is about obtaining the optimal process flowsheeting and conditions to achieve the desired products with the lowest capital and operating costs as well as minimal footprint such as plot space, various emissions and wastes, hydrogen, water, and energy. When all these are integrated seamlessly, the true optimal process design can be achieved for practical applications, which will benefit the companies, communities, and environment at the same time.
The cornerstone of this novel approach is the multiscale modeling and optimization integration via integrating fundamentals with the power of system integration and optimization, more specifically, integrating molecular analysis, quantum chemistry and transport mechanics with process integration and mathematical optimization for clean process development. By incorporating fundamentals into process development, it can identify the best molecular transformation routes by optimizing reaction pathways. Furthermore, mechanistic kinetic modeling such as microkinetic modeling and molecular-based kinetic Monte Carlo, which is enhanced by quantum chemistry, can help predict yield selectivity for different catalyst formula. By process integration, fit-for-purpose technologies are selected for developing hybrid systems. By mathematical optimization based on fundamental models, it can identify technological breakthrough ideas. For given multiple objective functions, mathematical combinatory optimization of the fundamental models not only determines the best-fit technology profile for an overall process based on techno-economic criteria, but also allow the process to deal with different feedstocks and make product shift based on market needs as well as the best environmental performance. In turn, this approach enables more effective feedback from development to research, as it integrated molecular based fundamentals.
When all these are integrated seamlessly, the true optimal process design can be achieved for practical applications, which will benefit the companies, communities, and environment at the same time.
To AI or Not to AI, is Part of the Loaded Question
Chris Clayton, Beyond Limits; Kim Gilbert, Beyond Limits
Artificial Intelligence (AI) is a broad field of endeavor with different definitions depending on who you are talking to, what you are reading or viewing. On a daily basis, nearly every aspect of our lives is touched, driven, or influenced by AI in some way, shape or form.
In our professional lives, we are challenged with how to make the best use of this incredible technology to improve our processes, products, experiences, and the value of our businesses. The question isn’t whether we should use AI. Our biggest challenge is knowing when and where to use it, how to scale it and at what cost? Another question, and perhaps the more important one, is when NOT to use AI or accept when we it cannot be used?
This session will discuss different AI approaches, techniques, and methodologies used within the process industry, as well as when to use them, and how to care and feed them over time. Note, the care and feeding applies to the systems we design and build and the vast array of incredibly smart people who brought them into reality.