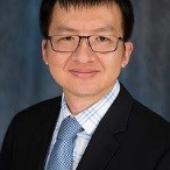
Data analytics is a key component of the digital transformation that is happening right now in the chemical industries. While collecting data has become easier with the development of technologies and software, the future of process development lies in the ability to understand and draw meaningful and predictive solutions via advanced analytic tools such as machine learning and artificial intelligence. Going beyond describing what happened or detecting what will happen in a chemical process, data analytics can provide guidance on the design, safety, and prevention of process upsets. This session will showcase examples of innovations in data analytics and their impact on process development.
Session Chairs:
- Mrunmayi Kumbhalkar, Dow
- Thu Vi, Merck
Schedule:
TIME (EDT) | PRESENTATION | SPEAKER |
---|---|---|
1:00 PM | Accelerating Multiscale Process Design with Bayesian Optimization: Progress, Challenges, and Opportunities | Joel Paulson, Ohio State University |
1:30 PM | Use of Advanced Multivariate Analysis to Solve Plant Problems | Sweta Somasi, Corteva Agriscience |
2:00 PM | Facilitate Process Optimization using Machine Learning Methodologies | Zhenyu Wang, Dow |
Abstracts:
Accelerating Multiscale Process Design with Bayesian Optimization: Progress, Challenges, and Opportunities
Joel Paulson, Ohio State University
Design problems, which are pervasive in science, engineering, and manufacturing endeavors, can be generally formulated as mathematical optimization problems. In ideal situations, one is able to develop a physics-based model of the system of interest whose structure can be exploited by state-of-the-art solvers, especially in cases that the derivatives can be exactly computed. However, the development of multiscale process models for which derivative information is readily available remains a significant challenge in many real-world applications. A particularly challenging class of problems is when at least one component of the model is very expensive or time-consuming to evaluate such as high-fidelity computer simulations (e.g., thermodynamic calculations, molecular dynamic simulations, or solutions to partial differential equations) and laboratory experiments (e.g., measurement of critical material, chemical, or biological properties). Although “black-box” optimization methods can be applied in these situations, many of the available algorithms require extensive sampling and thus are not tractable for expensive-to-evaluate objectives and constraints.
Recently, Bayesian optimization (BO) has emerged as a powerful tool for optimizing expensive black-box functions due to its successes in material/drug design and hyperparameter optimization in machine learning algorithms. In this talk, we provide an overview of BO and discuss its main advantages and disadvantages in the context of process systems applications. We also discuss two new advances in BO that can deliver considerable gains in performance by effectively “peeking inside the box” (i.e., selectively exploiting problem structure whenever possible), which we refer to as “grey-box” BO methods. In particular, we show how BO can be modified to handle composite functions and functions for which multiple lower-fidelity (cheap-to-evaluate) approximations are available. We then describe applications of these methods to (i) integrated design and control of flexible building heating and cooling systems with hourly variation in weather conditions over year-long simulations and (ii) calibrating genome-scale bioreactor models to experimental data.
Use of Advanced Multivariate Analysis to Solve Plant Problems
Sweta Somasi, Corteva Agriscience
Cross-functional collaboration of statisticians and chemical engineers can be a winning strategy to solve chemical plant problems. In modern chemical engineering plants, we collect massive amounts of data. This plant data is highly correlated due to the underlying mass and energy balance. Multivariate analyses, often referred to as chemometrics, have been used to analyze highly correlated data to solve plant problems. This data analysis combined with the right subject matter expertise can be a powerful strategy for troubleshooting. In this presentation, we will give an overview of multivariate analysis using a commercial tool (SIMCA). We will provide examples using actual plant troubleshooting problems. The examples will highlight how using these advanced data analytics methods, we were able to compare the stable plant operating conditions with the problematic ones and quickly identify the main differences. This led to identifying the exact root cause and quick resolution of the issue.
Facilitate Process Optimization using Machine Learning Methodologies
Zhenyu Wang, Dow
Process optimization is one of the key components for process development. Due to the complex nature of the industrial processes, especially (semi-) batches, process optimization usually relies on knowledge-driven models, which are developed based on first principles. In the last 50 years, the application of knowledge-driven models has increased considerably, especially for continuous chemical, petroleum, and petrochemical processes1. However, developing an accurate, knowledge-driven model is not always achievable. Some challenges that engineers/scientists may frequently encounter are: 1) lack of knowledge about process inner workings; 2) high cost to collect informative data to improve the model; 3) difficulty to justify the return from investment for model development; and 4) long development time of fundamental model to achieve the process optimization task.
As an alternative, machine learning methodologies (also known as data-driven methodologies) that optimize the process-based input-output relationships could be applied to optimize processes in a timely and economical manner. It has been proved that with a few well-designed experiments, similar optimal process performance could be achieved using a data-driven approach compared to the optimum obtained using knowledge-driven models2. The limitation of the data-driven methodologies is that their interpretability is lower compared to knowledge-driven approaches. So, leveraging the advantages of both data-driven and knowledge-driven approaches to facilitate the process optimization tasks is of high priority.
In this paper, we propose a hybrid methodology that combines an existing yet not very accurate knowledge-driven model with historical data collected from regular manufacturing activities to optimize the performance of a semi-batch process without running new experiments. Specifically, a Long-Short-Term-Memory (LSTM) network3,4 is developed using both simulated data from the knowledge-driven model and the historical data. The LSTM model learns the inner workings of the process described by both datasets. Then the LSTM network serves as an interactive environment to train the reinforcement learning (RL) agent5,6 to learn optimized fed-batch profiles. The RL agent suggests new optimal conditions with higher reactor temperature and higher reactant feed than the current ones. A 3% improvement in product values is suggested after implementing the optimal conditions in the knowledge-driven model. In addition, the estimated improvement also helps to justify the investment to further develop a more accurate and complete first principle model that could lead to higher process improvement.
References
1. Bonvin, D.; Georgakis, C.; Pantelides, C.; Barolo, M.; Grover, M.; Rodrigues, D.; Schneider, R.; Dochain, D., Linking models and experiments. Industrial & Engineering Chemistry Research 2016, 55 (25), 6891-6903.
2. Georgakis, C.; Chin, S.-T.; Wang, Z.; Hayot, P.; Chiang, L. H.; Wassick, J. M.; Castillo, I., Data-Driven Optimization of an Industrial Batch Polymerization Process Using the Design of Dynamic Experiments Methodology. Industrial & Engineering Chemistry Research 2020.
3. Hochreiter, S.; Schmidhuber, J., Long short-term memory. Neural computation 1997, 9 (8), 1735-1780.
4. Ma, Y.; Wang, Z.; Castillo, I.; Rendall, R.; Bindlish, R.; Aschraft, B.; Bentley, D.; Benton, M.; Romagnoli, J. A.; Chiang, L. In Reinforcement Learning-Based Fed-Batch Optimization with Reaction Surrogate Model, American Control Conference (ACC), New Orleans, Louisiana, USA, New Orleans, Louisiana, USA, 2021.
5. Schulman, J.; Wolski, F.; Dhariwal, P.; Radford, A.; Klimov, O., Proximal policy optimization algorithms. arXiv preprint arXiv:1707.06347 2017.
6. Rendall , R.; Ma, Y.; Castillo, I.; Wang, Z.; Peng, Y.; Chiang, L. In Applying Reinforcement Learning for Batch Trajectory Optimization in a Chemical Industrial Applicatio, AIChE Spring Meeting, Virtual, Virtual, 2021.