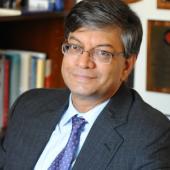
This session will cover the history of Industry 4.0 and how we got to where we are, and a second talk on how we transition to Industry 5.0 where we are aiming to get visionaries to explain how this transition will impact the industry.
Join us in welcoming Venkat Venkatasubramanian, Professor of Engineering, Columbia University, and Sam G. Samdani, Global Chemicals & Agriculture Practice, McKinsey & Company, as our plenary speakers for Industry 4.0!
Artificial Intelligence in Chemical Engineering: Quo Vadis?
Venkat Venkatasubramanian, Professor of Engineering, Columbia University
Artificial intelligence (AI) started off with great promise in the early 1980s, spurred by the success of the expert system paradigm in certain applications. This prompted a flurry of research activities in chemical engineering in the mid-1980s. However, as the ensuing three decades showed, AI didn’t quite live up to its early promise in chemical engineering.
So, what went wrong with AI?
In this talk, I will review the different phases of AI in chemical engineering in the last 35 years, providing some background and explanation to this question. I will also argue that this time it is different – I believe the time for AI in chemical engineering, and in other domains, has arrived, finally. There are many applications that are ready to yield quick successes in this new data science phase of AI. I will highlight recent work in materials design and in process operations as examples of exciting progress. However, the really interesting and intellectually challenging problems lie in developing such conceptual frameworks as hybrid models, mechanism-based causal explanations, domain-specific knowledge discovery engines, and analytical theories of emergence. These breakthroughs would require going beyond purely data-centric machine learning, despite all the current excitement, and leveraging other knowledge representation and reasoning methods from the earlier phases of AI. They would require a proper integration of symbolic reasoning with data-driven processing. I will discuss these challenges and opportunities going forward.
McKinsey on the Next Digital Leap to AI
Sam G. Samdani, Global Chemicals & Agriculture Practice, McKinsey & Company
Thanks to recent progress, breakthrough results and demonstrations of artificial intelligence (AI) capabilities in products and services already in wide use, there’s a lot of speculation that AI may usher in radical – arguably unprecedented – changes in the way people live and work. However, AI is not a single technology but a family of digital tools and techniques, e.g., computer vision, natural language, virtual assistants, robotic process automation, and advanced machine learning. Chemical companies are using these tools to varying degrees. Some are taking an opportunistic approach, testing only one technology and piloting it in a specific function. Others are much bolder, adopting all five and then absorbing them across their entire organization.
Based on an in-depth examination of more than 450 actual use cases of cross-industry application of AI, we found that AI has the potential to create annual value across sectors totaling $3.5 trillion to $5.8 trillion, or 40 percent of the overall potential impact from all analytics techniques. On average, our use cases suggest that modern deep learning AI techniques have the potential to provide a boost in value above and beyond traditional analytics techniques ranging from 30 percent to 128 percent, depending on the industry. The chemical industry, for example, stands to gain incremental value of 67% from deployment of AI over other analytics techniques.
Recent cross-industry research conducted by the McKinsey Global Institute (MGI) suggests that AI technologies could lead to a substantial performance gap between the front runners on one side and slow adopters and nonadopters on the other. At one end of the spectrum, front runners (i.e., companies that fully absorb AI tools across their enterprises over the next five to seven years) are likely to benefit disproportionately. By 2030, they could potentially double their cash flow (economic benefit captured minus associated investment and transition costs), which implies additional annual net cash flow growth of about 6 percent for more than the next decade. Front runners tend to have a strong starting digital base, a higher propensity to invest in AI, and positive views of the business case for AI.