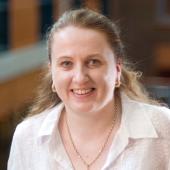
The first meeting of the American Institute of Chemical Engineers was held in Pittsburgh PA, December 1908. Our profession has seen dynamic and profound change in the 110 years since that inaugural meeting. This session will look at the future of chemical engineering through the eyes of thought leaders from industry, academia, and national laboratories.
Leading up to this event, AIChE presents "Revisiting the Future of Chemical Engineering," a blog series featuring predictions from these leaders in academia, industry, and government (national laboratories) on what the professional will be like 25 years in the future. This series reprises a similar effort completed for AIChE's Centennial Celebration ten years ago.
Overview
Chairs: Lorenz T. Biegler, Carnegie Mellon University and J. Karl Johnson, University of Pittsburgh
TIME | PRESENTATION | SPEAKER |
3:30pm | Welcoming Remarks | |
3:35pm | 25 by 25: Chemical Engineering in the Next 25 Years | Clare McCabe, Vanderbilt University |
4:03pm | The Future of Chemical Engineering Itself | Phil Westmoreland, North Carolina State University |
4:31pm | Accelerating Innovation through Academic-Industrial Partnerships | Bill Liechty, The Dow Chemical Company |
4:59pm | Maximizing Uptime, Efficiency, and Safety of Industrial Operations through Early Risk Detection | Ankur Pariyani, Near-Miss Management LLC |
5:27pm | Gaussian Processes for Hybridising Analytical & Data-driven Decision-making | Ruth Misener, Imperial College London |
5:55pm | Concluding Remarks |
Abstracts
25 by 25: Chemical Engineering in the Next 25 Years
Clare McCabe, Vanderbilt University
In 2008, the centennial year for AIChE, we asked 25 chemical engineers for their visions of the profession from around the US and the world: Nine faculty, eight from industry, and eight post-docs and graduate students. We posed four questions related to each individual's industrial sector or area of research, asking for extrapolation, new impacts on existing sectors, new sectors, and an optional, more open-ended comment on the future of the profession as a whole.
In this 110th year of AIChE, we have revisited these questions with many of the same people. Ten years has seen many developments in our profession, in society, and in our thought-leaders' experiences. Here we report on their perspectives, comparing them to the 2008 visions.
The Future of Chemical Engineering Itself
Phil Westmoreland, North Carolina State University
Chemical engineering’s future is bright because it is vital to so much of society and industry -- and its future is complicated by its rapidly expanding universe of activities. It must represent depth in core skills centered on the chemical sciences including biology and materials. At the same time, it must be transdisciplinary; it must exemplify the need and value of reaching across disciplines in borderless ways.
One way is to create and disseminate a compelling vision and strategy for chemical engineering, research, and education for the future. AIChE and the National Academies are working together to that end. In 1988, the National Academies published "Frontiers in Chemical Engineering: Research Needs and Opportunities.” Its committee of authors outlined a wide-ranging roadmap to turn promising research opportunities into reality, while calling for researchers to embrace new frontiers.
Since 1988, that has happened -- along with many other transformative changes like the Web, fracking, personalized medicine, and social media. A new nationwide effort, aided by international contributions, will address both the identity of chemical engineering and its future directions. Its goal is to ensure that the people in chemical engineering are primed to meet society's needs and rapid changes.
Accelerating Innovation through Academic-Industrial Partnerships
Bill Liechty* and Shawn Feist,The Dow Chemical Company
In 2011, The Dow Chemical Company launched the University Partnership Initiative (UPI) to sponsor collaborative research at leading U.S. Universities. This 10-year commitment aims to address fundamental questions in industrial chemistry and chemical engineering with alignment to Dow’s next-generation process technology and product development efforts. To date, this portfolio of projects has generated an impressive array of intellectual property, publications, and numerous examples of the collaborative developments implemented at Dow. This presentation will introduce UPI program and highlight several contributions from our collaborators and leaders in the chemical engineering profession.
Maximizing Uptime, Efficiency, and Safety of Industrial Operations through Early Risk Detection
Ankur Pariyani, Near-Miss Management LLC
Achieving zero incidents while maximizing process uptime and efficiency is a key vision for operating companies. An important building block towards this vision is establishment of a proactive risk mitigation culture, supported by effective systems and a strong management. Studies have shown that most of the incidents and unexpected process failures can be avoided if the operating teams get timely information about developing risks and take preventive actions early on. While advances have been made in process monitoring and automation in the past decades, there still remains significant technological and behavioral gaps that prevent plant operations from proactively mitigating process risks.
A modern industrial plant monitors thousands of parameters, generating upwards of 50-100 million data points every day. Thanks to recent breakthroughs in machine learning and artificial intelligence approaches, today there are autonomous systems that can sift through this data and point out meaningful and timely insight. This can help operating teams ascertain process issues that are hidden in the data, long before process variables reach critical levels.
In this presentation, a new autonomous system, Dynamic Risk AnalyzerTM (DRA), will be introduced that points out early indicators of process issues using proprietary machine learning. The risk indicators enable operations team to respond and make required process changes, days and sometimes weeks ahead of any alarm, avoiding late stage (expensive) fixes. To realize these objectives fully, a proactive management workflow must also be in place. The presentation will discuss new initiatives and workflows developed by plant operations to implement a proactive culture. Real-life case studies from plants using DRA technology will be presented on how this culture change resulted in increased uptime, efficiency and safety of plant operations.
Gaussian Processes for Hybridising Analytical & Data-driven Decision-making
Ruth Misener, Imperial College London
Surrogate models are widely appreciated in process systems engineering [1]. The typical setting focuses on expensive-to-evaluate, possibly uncertain functions. Examples include: modular process simulators [2], integrated gasification combined cycle processes [3], a carbon capture absorber [4], and many other applications, e.g. [5-8]. Resources are typically limited, so effective decision making requires data-efficient learning.
The data science and statistical machine learning communities typically focus on models learned solely from observed data. But chemical engineering applications may also require explicit, parametric models, e.g. modeling known process constraints, operations constraints, and cost objectives [9]. So, work has integrated semi-algebraic functions with those learned from data [10] or developed semi-physical modeling techniques [11, 12].
This presentation surveys the state-of-the-art in hybridizing analytical and data-driven decision making [13]. We consider three probabilistic modeling applications to these hybrid situations:
Design of experiments for model discrimination [14]. We bridge the gap between classical, analytical methods [15] and Monte Carlo-based approaches [16]. Classical methods may have difficulty managing non-analytical model functions and data-driven Monte Carlo approaches come at a high computational cost. We replace the original, parametric models with probabilistic, non-parametric Gaussian process surrogates learned from model evaluations. The surrogates are flexible regression tools that extend classical analytical results to non-analytical models, while providing us with model prediction confidence bounds and avoiding the computational complexity of Monte-Carlo approaches.
Multi-objective optimization [17, 18]. We make novel extensions to Bayesian multi-objective optimization in the case of one analytical objective function and one black-box, i.e. simulation-based, objective function. The resulting method has been applied to a bone neotissue application [19] and a more general test suite.
Scheduling plant operations under uncertainty. For processes with equipment degradation, we use Gaussian processes to approximate large-scale, mixed-integer optimization problems.
We close by offering a broad outlook on applying probabilistic surrogate models to chemical engineering. Statistical machine learning has recently attracted significant interest in process systems engineering [20]. Here we show that state-of-the-art research in Gaussian processes [21, 22] and probabilistic modeling more generally [23] can have a big impact on chemical engineering.
References
[1] Bhosekar A, Ierapetritou M. Advances in surrogate based modeling, feasibility analysis and and optimization: A review. Comput Chem Eng. 108: 250-267, 2018.
[2] Caballero JA, Grossmann IE. An algorithm for the use of surrogate models in modular flowsheet optimization. AIChE J. 54(10):2633-2650, 2008.
[3] Lang Y, Zitney SE, Biegler LT. Optimization of IGCC processes with reduced order CFD models. Comput Chem Eng. 35(9):1705-1717, 2011.
[4] Cozad A, Sahinidis NV, Miller DC. Learning surrogate models for simulation-based optim-ization. AIChE J. 60(6):2211-2227, 2017.
[5] Boukouvala F, Ierapetritou M. Surrogate-based optimization of expensive flowsheet modeling for continuous pharmaceutical manufacturing. J Pharm Innov. 8: 131, 2013.
[6] Soepyan FB, Cremaschi S, Sarica C, and others. Estimation of percentiles using the Kriging method for uncertainty propagation. Comput Chem Eng. 93:143-59, 2016.
[7] Shokry A, Ardakani MH, Escudero G, Graells M, Espuña A. Dynamic Kriging-based fault detection and diagnosis approach for nonlinear noisy dynamic processes. Comput Chem Eng. 106:758-76, 2017.
[8] Tran AP, Georgakis C. On the estimation of high-dimensional surrogate models of steady-state of plant-wide processes characteristics. Comput Chem Eng. DOI 10.1016/j.comp-chemeng.2018.02.014, 2018.
[9] Boukouvala F, Hasan MF, Floudas CA. Global optimization of general constrained grey-box models: new method and its application to constrained PDEs for pressure swing adsorption. J Glob Optim. 67(1-2): 3-42, 2017.
[10] Boukouvala F, Floudas CA. ARGONAUT: AlgoRithms for Global Optimization of coNstrAined grey-box compUTational problems. Optim Lett. 11(5): 895-913, 2017.
[11] Pearson RK, Pottmann M. Gray-box identification of block-oriented nonlinear models. J Process Contr. 10(4):301-315, 2000.
[12] Cozad A, Sahinidis NV, Miller DC. A combined first-principles and data-driven approach to model building. Comput Chem Eng. 73: 116-27, 2015.
[13] Boukouvala F, Misener R, Floudas CA. Global optimization advances in mixed-integer nonlinear programming, MINLP, and constrained derivative-free optimization, CDFO. Eur J Oper Res. 252: 701-727, 2016.
[14] Olofsson S, Deisenroth MP, Misener R. Design of Experiments for Model Discrimination Hybridising Analytical and Data-Driven Approaches. arXiv:1802.04170. 2018.
[15] Buzzi-Ferraris G, Forzatti P, Emig G, Hofmann H. Sequential experimental design for model discrimination in the case of multiple responses. Chem Eng Sci. 39(1): 81-85, 1984.
[16] Vanlier J, Tiemann CA, Hilbers PAJ, van Riel NAW. Optimal experiment design for model selection in biochemical networks. BMC Syst Biol, 8(20), 2014.
[17] Olofsson S, Mehrian M, Geris L, Calandra R, Deisenroth MP, Misener R. Bayesian Multi-Objective Optimisation of Neotissue Growth in a Perfusion Bioreactor Set-Up. Computer Aided Chemical Engineering. 40: 2155-2160, 2017.
[18] Beykal B, Boukouvala F, Floudas CA, Pistikopoulos EN. Optimal design of energy systems using constrained grey-box multi-objective optimization. Comput Chem Eng. DOI 10.1016/j.compchemeng.2018.02.017, 2018.
[19] Mehrian M, Guyot Y, Papantoniou I, Olofsson S, Sonnaert M, Misener R, Geris L. Maximizing neotissue growth kinetics in a perfusion bioreactor: An in silico strategy using model reduction and Bayesian optimization. Biotechnol Bioeng. 115(3):617-29, 2018.
[20] Lee JH, Shin J, Realff MJ. Machine learning: Overview of the recent progresses and implications for the process systems engineering field. Comput Chem Eng. DOI 10.1016/j.compchemeng.2017.10.008, 2017.
[21] Damianou A, Lawrence N. Deep Gaussian processes. In Artificial Intelligence and Statistics. 207-215, 2013.
[22] Deisenroth MP, Ng JW. Distributed Gaussian processes. In 32nd International Conference on International Conference on Machine Learning. 37: 1481-1490, 2015.
[23] Ghahramani Z. Bayesian non-parametrics and the probabilistic approach to modelling. Phil Trans R Soc A. 371(1984):20110553, 2013.