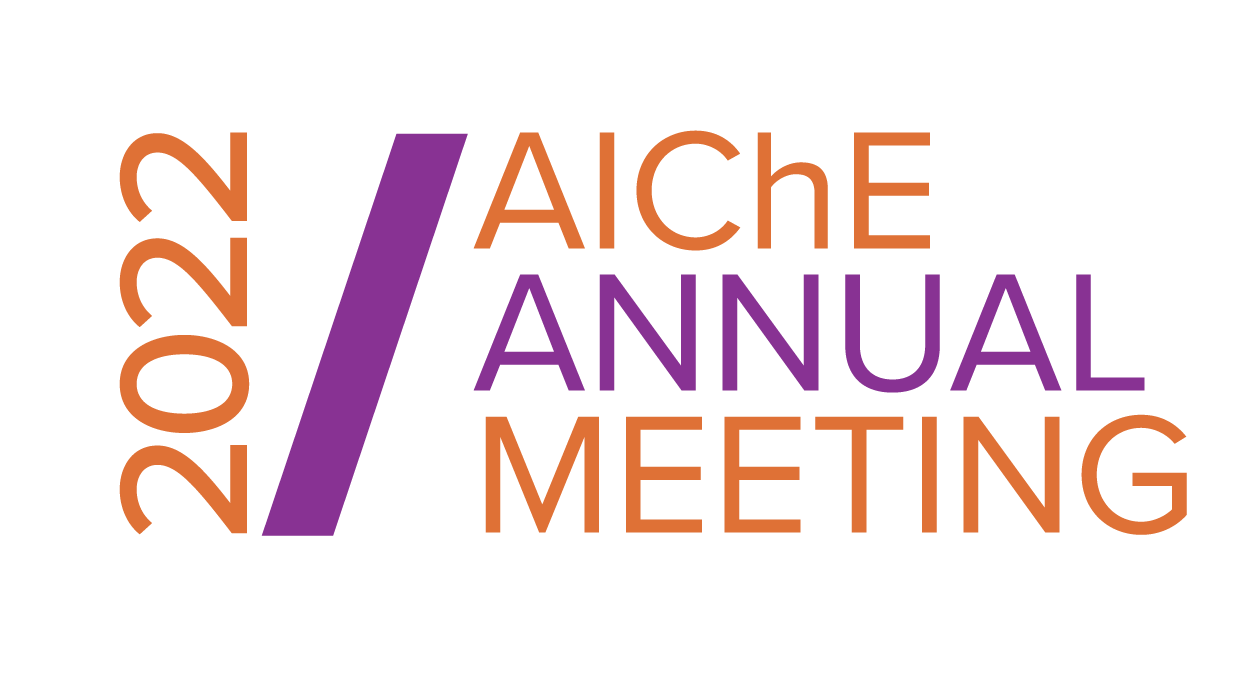
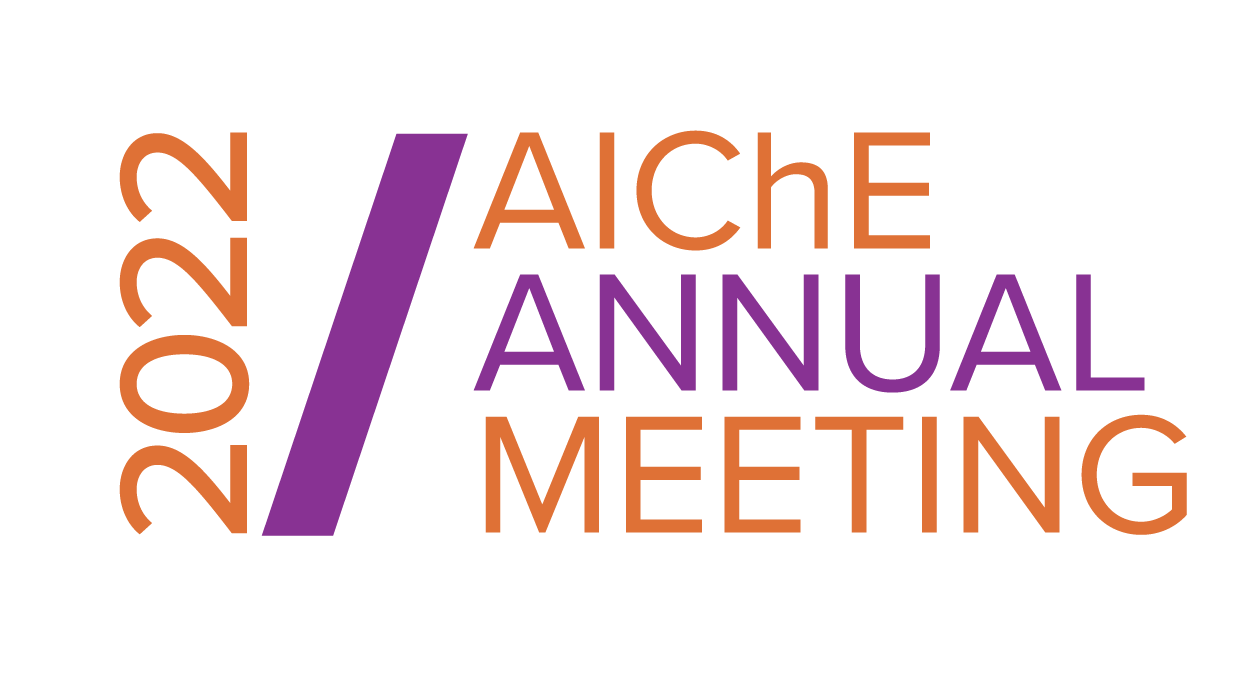
Metal nanoparticles find tremendous application in modern technology, ranging from catalysis to medicine. However, how different metals mix at the nanoscale and their exact thermodynamically preferred chemical ordering (positioning of different metal atoms on the nanoparticle lattice) is unknown. In this presentation, I will highlight recent computational advances on accurately describing the thermodynamic stability of bimetallic nanoparticles of any size, shape, metal composition and chemical ordering, by combining Density Functional Theory calculations with machine learning. The developed models can be extended to multimetallic nanoparticles, as well as used for the description of chemical adsorption on the nanoparticle surface, thus, connecting nanoparticle stability with application properties (sensing, catalysis, etc.).
Presenter(s)
Once the content has been viewed and you have attested to it, you will be able to download and print a certificate for PDH credits.
If you have already viewed this content,
please click here
to login.