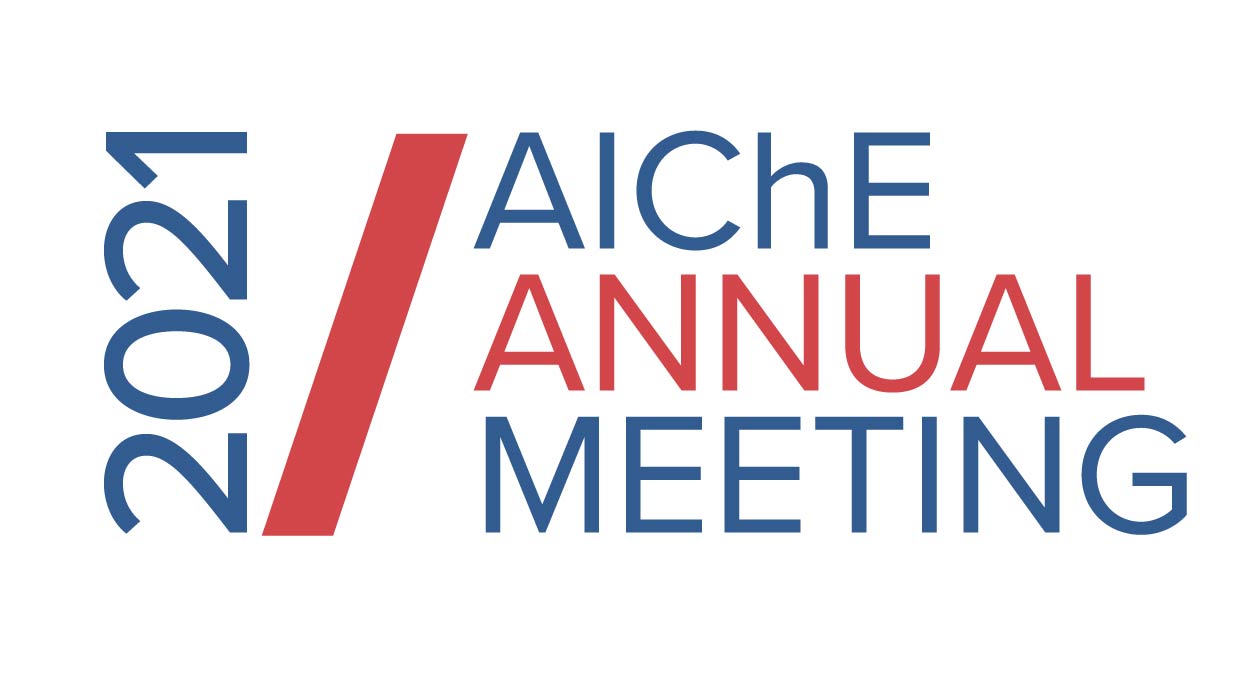
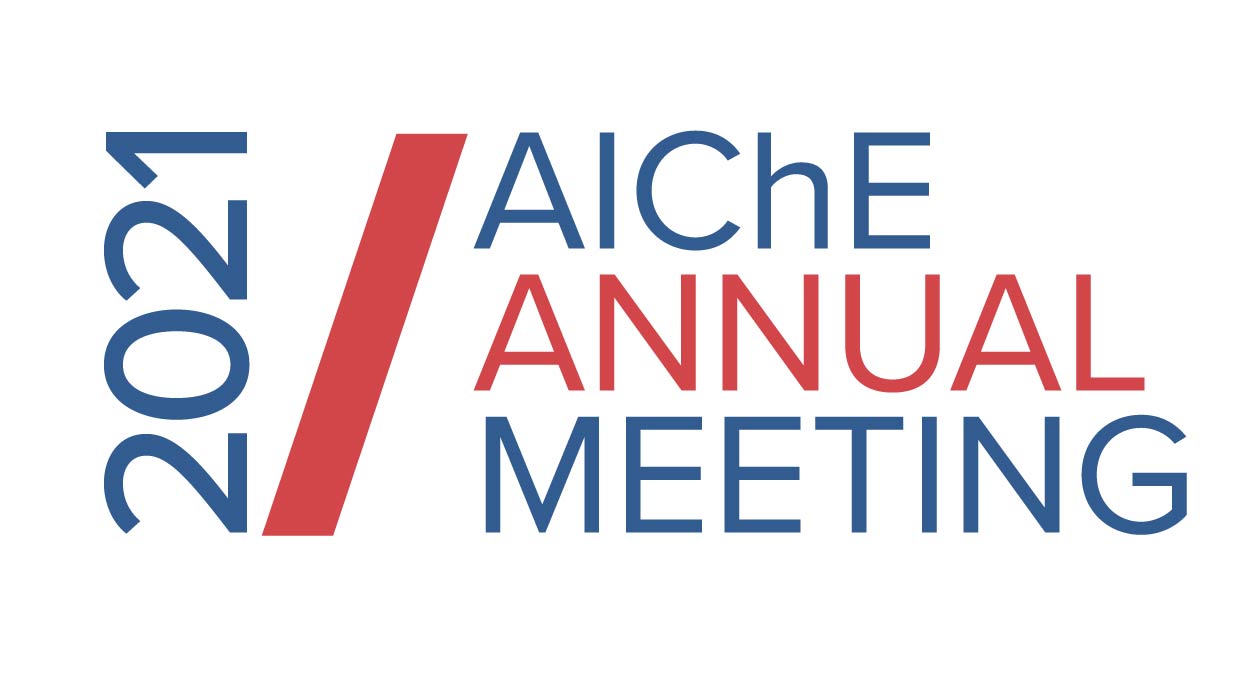
The health and performance of a chemical system deteriorates rapidly during its lifetime due to a variety of possible factors. These factors include possible wear and tear, extreme environmental conditions, human error, improper usage of the system or possible occurrence of process abnormalities. If these wear or damages to a system are not detected at an early stage, they will cumulatively increase in a slow or rapid manner (depending on the dynamics of the process) and can lead to plant shutdown, loss of process downtime, loss of revenue and in the worst case, unexpected accidents, catastrophes and human safety lawsuits against the investing companies using these chemical systems for their business and product development purposes.[1]
In an era where competitiveness and profits are dependent on a companyâs ability to produce highly dependable systems and products for their customers, the reliability, security, longevity and availability of their processing/operating systems must be ensured. That is, optimal maintenance strategies must be employed to draw out an accurate assessment of the systemâs condition and performance in order to determine the type, kind and frequency of maintenance.
So far, industrial maintenance of chemical systems have largely been manual and dependent on an operatorâs understanding and knowledge of the systemâs evolving dynamics. Most maintenance strategies have been intuitive in nature and dependent on the personnelsâ experience, knowledge and familiarity with the chemical system. Earlier maintenance techniques were in the form of reactive maintenance and scheduled maintenance, where in maintenance was only performed when the system ran to a state of complete failure or at fixed intervals of time. However due to fears regarding the potential costs of repair, possibility of failure in between shutdowns and the downtime involved with these maintenance actions, companies have had to rethink their long-followed heuristic maintenance patterns and search for more beneficial techniques that can help them reduce their large investments in maintenance planning.
Predictive or Condition-Based Maintenance (CBM) is now seen as the most promising approach for ensuring the reliability and safety of a system. Unlike the previously established approaches which ignored the wealth of data collected from a process during its operation, the CBM strategy utilizes the available system monitoring data to firstly provide a holistic view of the current state of a system and then trigger potential warnings for plant engineers to pre-plan their maintenance strategy, well in advance, to avoid any disruptions or temporary halts in the system operation. This approach reduces the potential costs of maintenance and repair, and also ensures the safety and reliability of a system. With advancements in data collection technology, improved processing powers of prognostic algorithms and readily available pool of highly reliable sensors, the field of maintenance has moved from a feedback approach to a feed-forward approach. CBM is a key concept of this Industry 4.0 revolution.
Fault Diagnostics (FD) and Fault Prognostics (FP) are the two most crucial components of the CBM strategy. Using the available condition data collected from a system, these components are firstly used to detect the onset of a system abnormality and on occurrence of said abnormality, estimate the remaining useful lifetime (RUL) of a system so that predictive maintenance can be planned in advance or before the system reaches the state of absolute failure.[2]
In this paper, an integrated data-driven fault diagnostic-prognostic approach for the health condition monitoring of degrading systems is proposed. Most of the past research in this area has been system-specific and a generalized data driven approach for the health condition monitoring of systems has not been developed yet. Furthermore, past research has focused on the implementation of approaches based on on commonly available simulated, artificial or experimental data. There has been no research so far that has focused on the online implementation of a fault diagnostic approach for realistic and noisy industrial data. As researchers spend a lot of time in finding the best model for their specific purpose, the development of a general library of data driven models for FD and FP purposes would be helpful in saving their valuable time, energy and efforts. This paper aims at addressing these gaps in the literature.
Although many types of models such as 1) Physics-based or First-Principles-based models 2) Data-driven models and 3) Hybrid models have been developed and utilized for FD and FP models, machine learning data driven models have been utilized for our proposed library due to their relative simplicity of development and good levels of model accuracy. Furthermore, as the dynamics and mechanistic process behaviour varies from system to system, it is difficult to develop a generalized first-principles-based model approach applicable to all kinds of chemical systems. Therefore, data driven models infused with system specific knowledge (or) hybrid models are seen as the best possible ways of building of such a generalized approach for FD and FP purposes.[3]
Our proposed approach involves a library of machine learning data-driven classification and regression models, wherein the library of classification models is first used to train and provide the best performing classifier model to detect and isolate the faults occurring in a system. On accurately identifying the first occurrence of the fault or the First Predicting Time (FPT) of failure, the second step involving a library of Multi-Input Single Output (MISO) regression models is used to provide the best regression model to estimate the RUL. The reason behind the selection of MISO regression over Single Input Single Output (SISO) regression models is because most chemical processes are generally multivariate in nature, wherein multiple process variables can affect the health and state of a system and its dynamics.
The members of our proposed classification and regression library include Support Vector Machines (SVMs) (with polynomial, Gaussian and linear kernel), Regression Trees (RT) ensembles and Artificial Neural Networks (ANN) (with RELU and Tansig activation functions). Although feature extraction is an important part of finding the most appropriate process variables or health indicators of a system, the proposed approach only deals with finding the best classifier and best regressor for fault detection and fault prognostic purposes, when given the ideal health indicators of a system.
As the availability of simultaneous FD and FP data for a single system is limited, the accuracy of the individual libraries of the proposed approach is tested and assessed with the help of a series of simulated and real industrial case studies. The proposed classification and regression library approach is firstly tested and assessed on the publicly available classification datasets such as the CNC milling dataset and the triplex pump dataset (from MATLAB) for FD purposes as well as the commonly available PHM Challenge regression datasets such as the Turbofan Engine dataset and Battery degradation dataset for FP purposes. Furthermore, in order to further validate its real life and online implementation for industrial systems, the proposed approach was tested on a system of Booster pump mesh filters for both FD and FP purposes. Based on the conducted testing and analysis, the best classifier and regression model (which had the least test error performance) was obtained for each dataset and its classification or regression error performance was compared with its fellow member models as well other published works on these datasets. It was found that our results in all cases were in agreement with the results obtained from other such works on these datasets, which demonstrates the success and efficacy of our proposed approach in the health condition monitoring of degrading systems.
Keywords: Health monitoring, Fault Detection & Diagnostics (FDD), Remaining Useful Lifetime (RUL) prediction.
REFERENCES:
[1] A. K. S. Jardine, D. Lin, and D. Banjevic, âA review on machinery diagnostics and prognostics implementing condition-based maintenance,â Mechanical Systems and Signal Processing, p. 28, 2006.
[2] Y. Lei, N. Li, L. Guo, N. Li, T. Yan, and J. Lin, âMachinery health prognostics: A systematic review from data acquisition to RUL prediction,â Mechanical Systems and Signal Processing, vol. 104, pp. 799â834, May 2018, doi: 10.1016/j.ymssp.2017.11.016.
[3] A. Heng, S. Zhang, A. C. C. Tan, and J. Mathew, âRotating machinery prognostics: State of the art, challenges and opportunities,â Mechanical Systems and Signal Processing, p. 16, 2009.