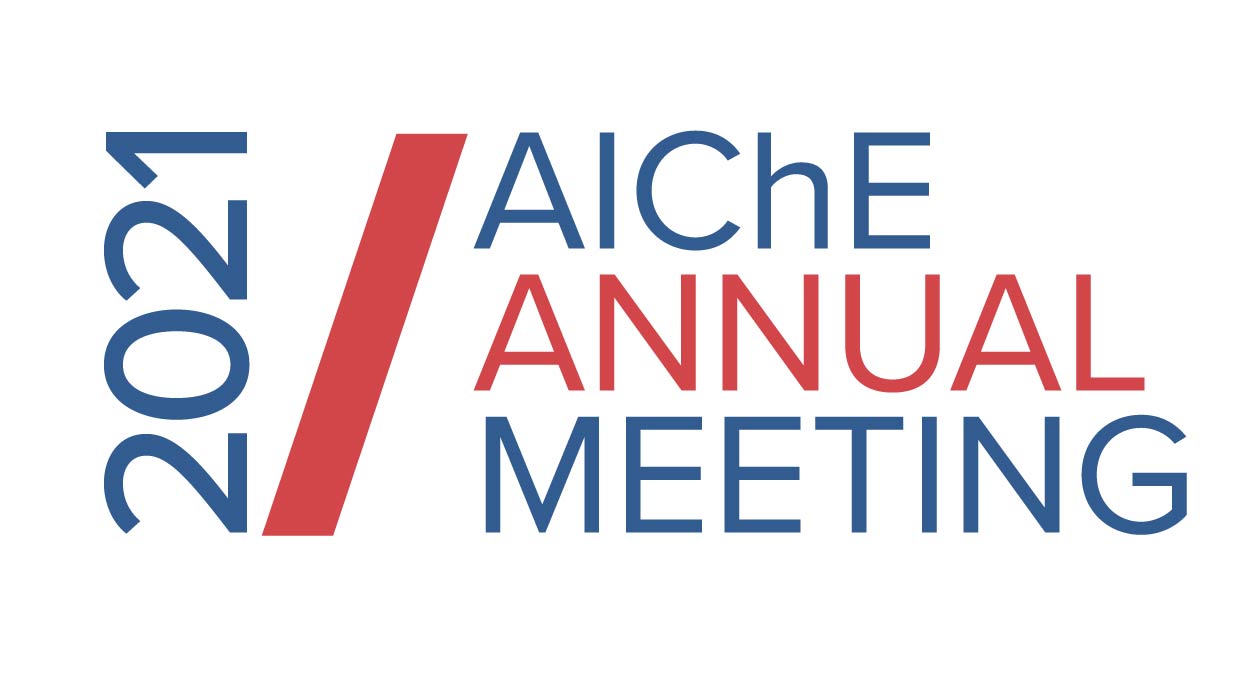
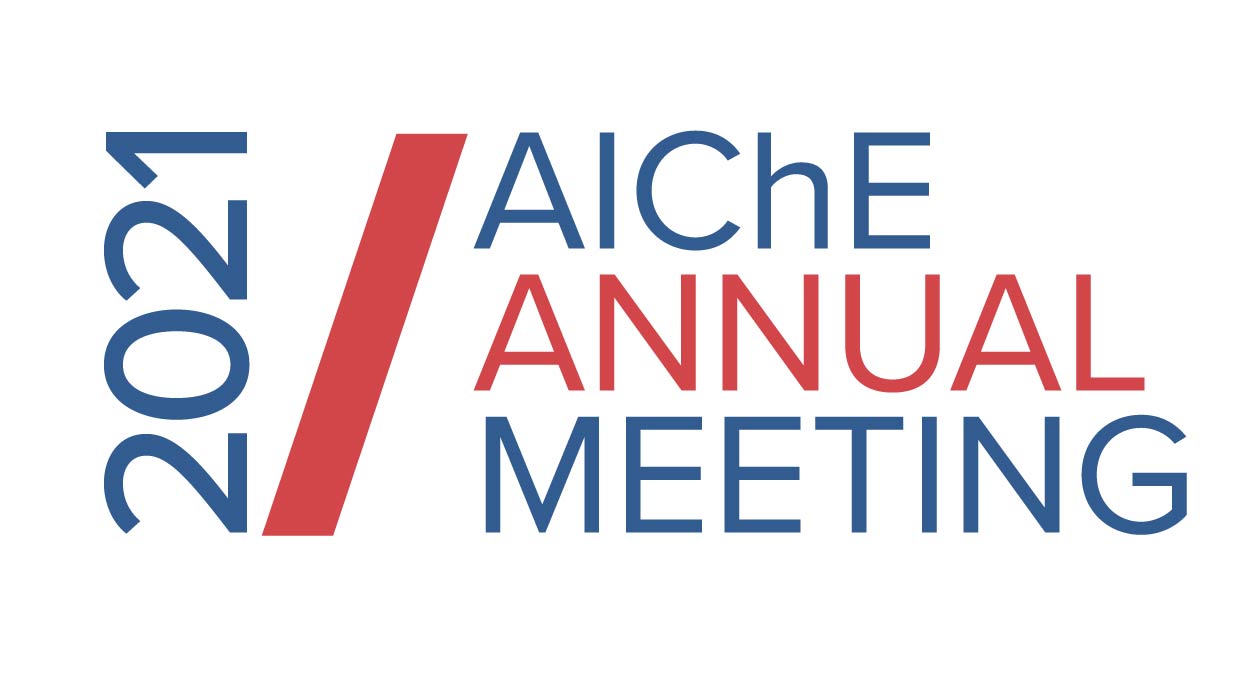
Here we present a data-driven coarse-graining methodology for discovering such emergent, reduced models. We consider two types of reduced models: globally-based models which use global information and predict dynamics using information from the whole ensemble, and locally-based models that use local information, that is, information from just a subset of agents close (close in heterogeneity space, not physical space) to an agent, to predict the dynamics of an agent. For both approaches we are able to learn laws governing the behavior of the reduced system on the low-dimensional manifold directly from time series of states from the agent-based system. These laws take the form of either a system of ordinary differential equations (ODEs), for the globally-based approach, or a partial differential equation (PDE) in the locally-based case. For each technique we employ a specialized artificial neural network integrator that has been templated on an Euler time stepper to learn the laws of the reduced model. As an illustration of the efficacy of our techniques, we consider a simplified all-to-all coupled neuron model for the rhythmic oscillations in the pre-Bötzinger complex and demonstrate how our data-driven surrogate models are able to produce dynamics comparable to the dynamics of the full system. An interesting consequence of our PDE methodology is that our local PDE model is able to learn the behavior of a globally coupled model. This suggests that the neural network learns a coupling topology, different from the actual coupling topology of the agents, that is conducive to modeling the dynamics, although this topology is not directly accessible.
[1] Y. Kuramoto, âChemical Oscillations, Waves and Turbulence,â Synergetics 19 (1984).
[2] S. Watanabe and S. H. Strogatz, âIntegrability of a globally coupled oscillator array,â Physical review letters 70, 2391 (1993).
[3] K. P. OâKeeffe, H. Hong, and S. H. Strogatz, âOscillators that sync and swarm,â Nature communications 8, 1â13 (2017).
[4] S. H. Strogatz, âFrom Kuramoto to Crawford: exploring the onset of synchronization in populations of coupled oscillators,â Physica D: Nonlinear Phenomena 143, 1â20 (2000).
[5] C. Bick, M. Goodfellow, C. R. Laing, and E. A. Martens, âUnderstanding the dynamics of biological and neural oscillator networks through exact mean-field reductions: a review,â The Journal of Mathematical Neuroscience 10, 1â43 (2020).
[6] E. Ott and T. M. Antonsen, âLow dimensional behavior of large systems of globally coupled oscillators,â Chaos: An Interdisciplinary Journal of Nonlinear Science 18, 037113 (2008).