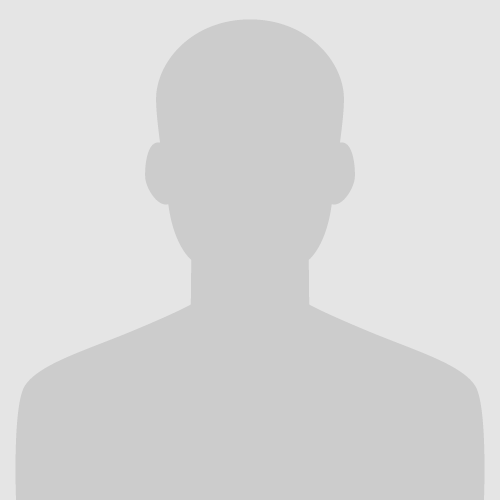
Session Chair
- David Couling, The Dow Chemical Company
Session Description
Computer simulation is a useful tool for many process development projects, and its utility will grow as computational power continues to increase. Process simulations can help predict product outputs, illustrate the interdependencies of key unit operations, demonstrate consequences of process upsets, and more. Moreover, process optimization routines can be used to mathematically determine optimal operating conditions rather than relying on heuristics or costly experimentation. This session focuses on both process simulations and optimization routines that have been developed and used in order to improve process performance.
*All session and speaker information is subject to change pending finalization
Schedule:
TIME | PRESENTATION | SPEAKER |
8:55am | Comparison of Modeling Approaches to Optimize a District Heating & Cooling Facility | David Hill, Chemstations |
9:25am | ALAMO: Machine Learning from Data and First Principles | Zachary Wilson, Carnegie Mellon |
9:55am | The Practice of Simulation in Process Engineering and Optimization | Kheng Lau, BP |
Abstracts:
Comparison of Modeling Approaches to Optimize a District Heating & Cooling Facility
David Hill, Chemstations
Azbil is optimizing setpoints for a district heating-cooling facility. A commercial process simulator (CHEMCAD) is being used to model the facility operation. The model is first validated against historical performance, and then used to recommend new setpoints online.
A building in Yokohama supplies chilled and heated water to several neighboring buildings in the office district. User demand and the weather are difficult to predict. The building has the option to turn on/off several cooling towers and multiple refrigeration units. Substantial cost savings can be achieved by changing the number of active units to minimize the power used by the pump networks, cooling tower fans, and refrigeration networks.
Previously an LP (linear programming) optimization model was developed for this facility using process control concepts (gain, transfer functions, black box, etc.). Azbil is developing a model which uses a commercial process simulator to model the process. Using a commercial process simulator reduces the amount of time to develop the model, and increases the accuracy of the model used to determine optimized setpoints.
ALAMO: Machine Learning from Data and First Principles
Zachary Wilson, Carnegie Mellon University
Models of systems and processes are routinely used to facilitate design, optimization, and intelligent decision making. The increasing abundance and availability of large and descriptive data sets has increased the use of data-driven models to aid in these tasks. ALAMO is a computational methodology for the data-driven learning of algebraic models. Through explicit parametric transformations of system inputs, and linear selection of model features, ALAMO is capable of building models tailored for use in equation oriented optimization algorithms. Insights obtained from first principles or modeler insight can be applied directly to modeled responses using constrained regression, a semi-infinite programming approach for enforcing response constraints in the space of the model coefficients.
The capabilities of ALAMO are demonstrated through a number of case studies. Alternative data-driven model building methodologies are compared through the optimization of the resultant models. A linear model selection algorithm using a combination of search heuristics, a regularization filter, and the final integer optimization of a model fitness metric provides efficient selection of predictive models. New data is acquired through an adaptive design of experiments, error maximization sampling, in order to efficiently sample a system and certify model quality. Benchmark data sets are used to compare the model selection and adaptive sampling algorithms used by ALAMO against alternative approaches.
The Practice of Simulation in Process Engineering and Optimization
Kheng Lau, BP
Sequential modular and equation-based simulation technologies are used widely nowadays. Both of these technologies play significant roles in steady state and dynamic simulation. Process simulation software has evolved in the last 20+ years. It is certainly easier to develop for example a distillation column model that matches process data fairly closely today. Advances in computing hardware and industrial standards have certainly transformed how simulation work is done. Process simulation enables many engineers around the world analyze and solve many engineering problems that they encounter. In addition, engineers use process simulation to evaluate new ideas in improving a process design or running a process unit. The process model used in simulation work must meet requirements defined for the intended application. Steady state and dynamic simulation application examples will be shared to illustrate important advances in simulation technology that make these applications possible.