(197az) Dataset and Models for Predicting Critical Properties of Fluids
AIChE Annual Meeting
2023
2023 AIChE Annual Meeting
Computational Molecular Science and Engineering Forum
Poster Session: Computational Molecular Science and Engineering Forum
Monday, November 6, 2023 - 3:30pm to 5:00pm
Knowledge of critical properties, such as critical temperature, pressure, density, and acentric factor, is essential to calculate many different thermodynamic properties of chemical compounds. The values of critical properties are also used in models for mixtures, including for predicting temperature-dependent solvation energies and solubilities. Experiments to determine critical properties are expensive and time intensive, so it is desirable to glean as much information as possible from that hard-won experimental data. Therefore, we compiled/curated a data set of critical property values on 916 pure chemical compounds, and used that data to develop a machine learning (ML) model that can predict critical properties given as input the SMILES representation of a neutral chemical species. We explored directed message passing neural network (D-MPNN) and graph attention network as ML architecture choices. Additionally, we investigated featurization with additional atomic and molecular features, multi-task training, and pre-training using estimated data to optimize model performance. Our final model utilizes a D-MPNN layer to learn the molecular representation and is supplemented by Abraham parameters estimated using methods in the literature. A multi-task training scheme was used to train a single model to predict all the critical properties simultaneously with boiling point, melting point, enthalpy of vaporization, and enthalpy of fusion. The model was evaluated on both random and scaffold splits where it shows state-of-the-art accuracies. The extensive critical property data set containing 916 chemical compounds are made available in the public domain along with the model source code that can be used for further exploration.
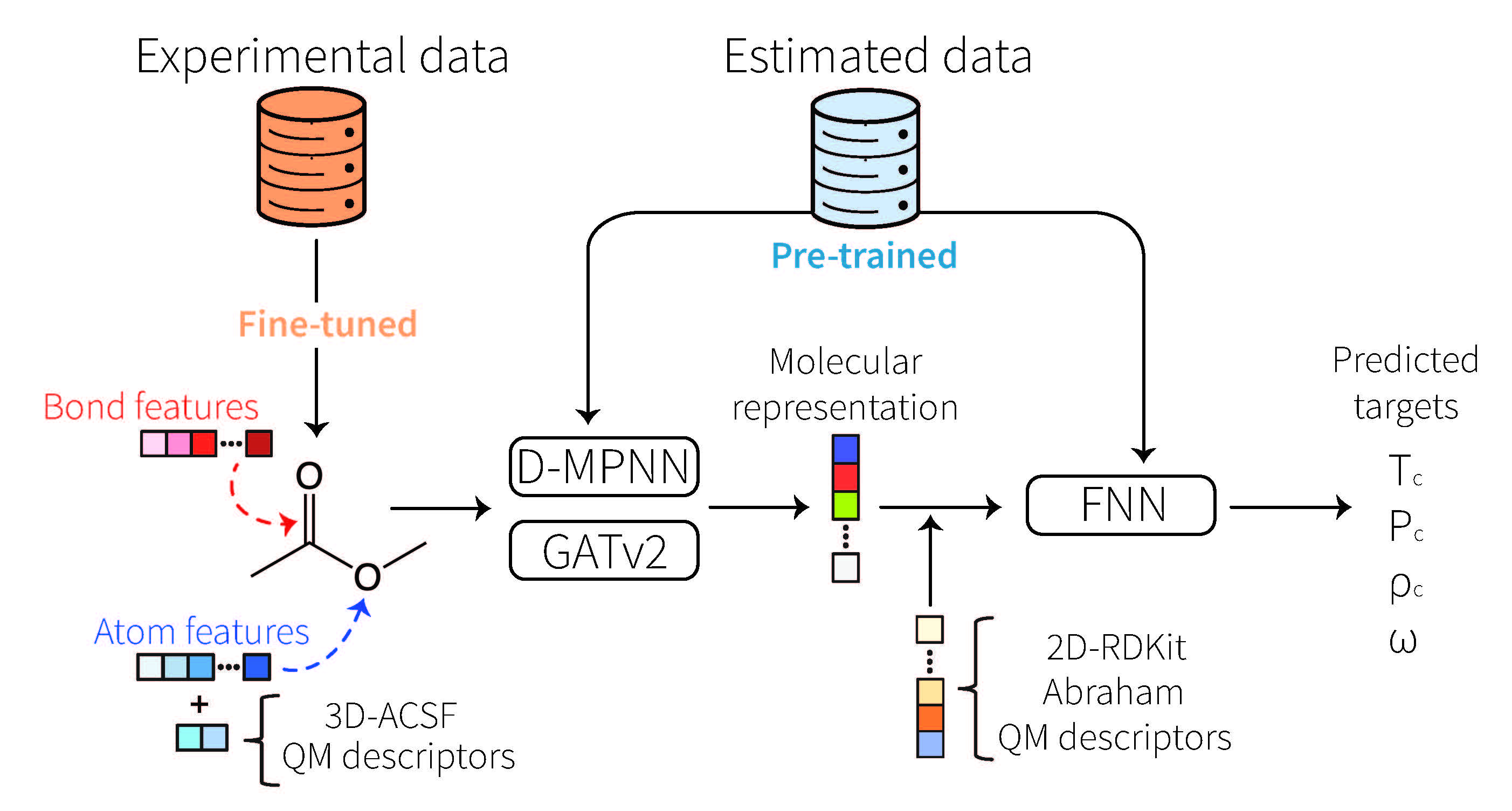
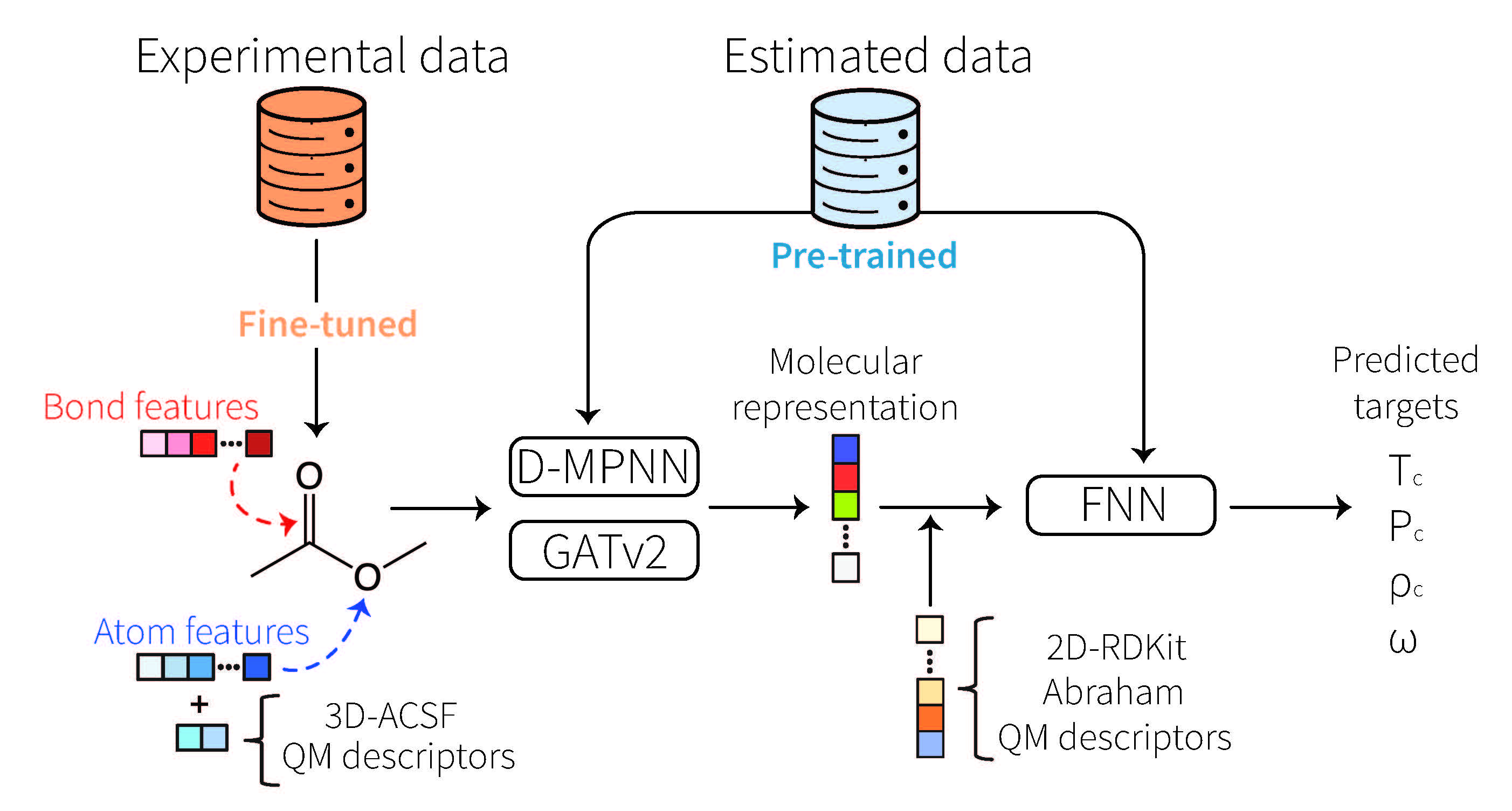