The chemical process industries (CPI) are hurtling toward a smarter future, but confusion and exaggeration around new technologies are causing some to want to stay behind. Sober guidance on selection, implementation, and utilization of digital tools can help differentiate the hope from the hype.
Terms such as digitalization, big data, and artificial intelligence (AI) have become ubiquitous in the past few years. Control system vendors, software solutions providers, and industry consultants use these terms to describe myriad offerings that they claim are driving the fourth industrial revolution (i.e., Industry 4.0) and will transform manufacturing into so-called smart manufacturing (SM). Executives hear about the benefits of big data, plant operators are promised predictive maintenance, and IT and R&D are up in a cloud and tangled in an internet of things (IoT), or so it seems.

▲Figure 1. Confusion around smart manufacturing is due to the many equivalent terms, new IT terms, and new underlying tools and technologies that are being applied in virtually every functional area.
Confusion abounds as these new terms and ideas are applied to familiar concepts (Figure 1). We are all left wondering: Are these claims real? What precisely is smart manufacturing? Why do so many terms seem to mean the same thing? Isn’t the chemical industry already automated enough? How can my company deal with this new wave of tools and technologies? This article attempts to answer these questions by defining some of these new terms and describing their relationships.
Smart manufacturing
Smart manufacturing is an evolving state of manufacturing in which digital solutions enable enhanced system connectivity, data analytics, and functional automation that improve operational agility, adaptability, and profitability. This definition encompasses smart manufacturing’s evolving nature, enabling systems (e.g., digital solutions such as the IoT and AI), improvement drivers (e.g., connectivity, analytics, and automation), and final outcomes (e.g., operational agility, adaptability, and profitability). Industry 4.0 is an alternative term for smart manufacturing that is used mostly in Europe and refers to the fourth industrial revolution.
The digital solutions that enable smart manufacturing are based on subsystems, including data collection and storage (e.g., smart measuring devices, databases), internet/intranet connectivity for data exchange (e.g., IoT, cloud), data analysis and decision-making (e.g., big data, analytics, AI), decision implementation mechanisms (e.g., control, automation), and human-machine interfaces (e.g., workstations, mobile phones).
Industrial internet of things. A primary characteristic of smart manufacturing is that its subsystems are connected and can exchange information in real time via the industrial internet of things (IIoT). The various “things” might include manufacturing equipment (such as process units, controllers, and machines), computers, software, human experts, and end-users. The term internet of things (IoT) is more general and extends beyond the realm of manufacturing.
To complicate things further, IoT and IIoT are sometimes referred to as cyber-physical systems (CPS), because things in an IoT/IIoT can be physical equipment or be computational- or web-based (cyber). Moreover, services provided via IoT/IIoT are sometimes called connected services.
Cloud. A cloud is a platform that provides data storage, computing, and communication hardware and software in place of conventional physical servers. The difference between a cloud and a server is that a server is usually owned by and dedicated to a single company, while a cloud can serve large numbers of companies and individuals, usually on a pay-per-usage basis. A cloud can be used for general-purpose storage and computing needs, or it can be used to host the IoT/IIoT. A small-scale IoT may be implemented via conventional servers.
The popularity of commercially available clouds can be attributed to their pay-per-usage scheme. Application developers that utilize a cloud do not need to invest upfront in computing infrastructure or maintain and update hardware and software, and it allows developers to easily scale their applications. Generic clouds from companies like Microsoft and Amazon enable software as a service (SaaS), platform as a service (PaaS), and infrastructure as a service (IaaS). Predix from GE, Sentience from Honeywell, and MindSphere from Siemens are examples of industrial clouds specifically designed for the manufacturing IIoT.
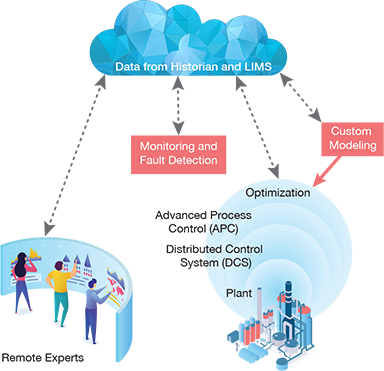
▲Figure 2. Connected services are enabled by data historians and laboratory information management systems (LIMS) that send real-time data via a cloud to remote experts. This gives these remote teams a view into plant operation and enables them to make recommendations.
Clouds have been recently used by process technology licensors to provide connected services using near-real-time operational data that is continuously collected and transmitted to a panel of remote technology models and experts. These experts analyze the data regularly and provide solutions for troubleshooting, asset reliability, and operational optimization (Figure 2). (UOP/Honeywell, for example, provides such systems and services under the brand name Connected Performance Services [CPS] for plants that leverage UOP technologies.)
Other tools. The IIoT and clouds are primarily mechanisms for data storage, data transfer, and connectivity. Predictive analytics, AI, and digital twins are another class of smart manufacturing tools that use all of the data gathered to make timely inferences and decisions, which helps to optimize operation in real time.
The data gathered is dubbed big data. These huge volumes (terabytes) of data can be processed and analyzed to gain insight into systems. Predictive analytics use big data to predict system behavior and trends.
Artificial intelligence is software-based cognitive capability that allows computers or machines to infer decisions based on rules and data. Some of the systems that apply AI use the term smart as a qualifier or prefix in their names or descriptions. Machine learning is one of the most relevant branches of AI in the context of smart manufacturing. It is a branch of AI that gives computers the ability to learn from data and can be incorporated in predictive analytics.
A digital twin of equipment is an adaptive model that is continuously updated to reflect the changing features of the physical object. Usually, a 3D visualization of a physical object is part of a digital twin model.
Digitalization: A means to achieve smart manufacturing
In an ideal IIoT, every entity would have up-to-date knowledge of properties and capabilities of other connected entities and would be able to manipulate their properties. Any entity in a manufacturing enterprise, technical or nontechnical, that can generate and communicate data can either benefit from digitalization or provide a benefit to other connected entities.
The question is now: What is digitalization and what is its relationship to smart manufacturing?
Digitalize means to convert a continuous (analog) signal into a discrete signal of binary digits. In automation, the term or prefix digital has been used to describe controllers and related instrumentation that use discrete signals, unlike older analog instrumentation based on analog (continuous) signals.
The term digitalization is now applied in a much broader and more generic sense. It is used to describe the implementation of any solution that involves computer hardware or software. Digitalization is an essential enabler of smart manufacturing, but it is sometimes used as a synonym. In such cases, it is used to describe a solution that connects system components (e.g., equipment, processes, instruments, analyzers, models, people) by enabling them to communicate via streams of digital data and to utilize that information.
Plant digitalization is not totally new to the chemical process industries (CPI). CPI facilities were among the early implementers of digital technologies for automation, safety, and optimization. The scope of digitalization, however, has expanded and involves cutting-edge technologies, including connectivity, mobility, predictive analytics, AI, and robotics. Digitalization in the CPI is enabled by:
- data connectivity through clouds. Plant operational data shared via a cloud can be used for various objectives, including near-real-time troubleshooting by remote technology experts.
- advanced process control (APC) and real-time optimization (RTO). APC and RTO help optimize plant operation, maintaining it within applicable constraints. APC uses dynamic models of individual units (e.g., reactors, distillation columns), while RTO uses rigorous steady-state models to perform a full plant flowsheet optimization.
- big data and predictive analytics. Operational data can be analyzed to predict imminent process upset or equipment faults. For example, analysis of vibration data can help to predict a fault in a compressor.
- robotics, smart sensors, radio-frequency identification (RFID), augmented and virtual reality, etc. Smart sensors not only measure the intended phenomenon but also report their own accuracy. Moreover, smart sensors themselves perform situation-specific preprocessing of measured signals. This improves measurement reliability and system uptime. Robotics can be used in difficult or dangerous jobs in various R&D or plant operations.
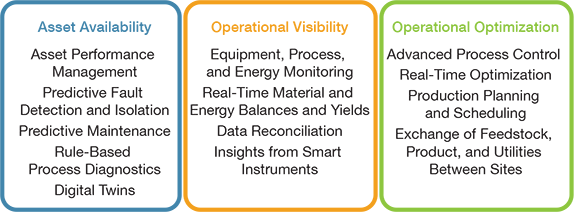
▲Figure 3. Digitalization can improve asset availability, operational visibility, and operational optimization.
A CPI company can leverage digitalization to improve asset availability, operation visibility, and operational optimization (Figure 3).
Big data
For decades, chemical plants have been collecting and archiving large amounts of structured operational data. A distributed control system (DCS) gathers and uses this data to monitor and control equipment and processes in real time. Process engineers routinely analyze data obtained through the DCS or plant historians via spreadsheets for plant troubleshooting and improvement. And, for more than two decades, plant data has also been used to develop artificial neural network (ANN) models for APC. (Read “Introduction to Deep Learning: Part 1,” in the June 2018 issue of CEP for more on ANNs.)
However, much plant data that is archived is seldom revisited. Availability of technical data is not the problem; rather, the problem is finding the time, tools, and expertise to analyze it. Nearly two-thirds of chemical executives rank big data as one of their top three concerns with respect to digital transformation (1).
Big data itself is nothing more than huge amounts of real-time and archived data. Distributed file systems (e.g., Hadoop HDFS) and distributed/parallel computing environments (e.g., Hadoop MapReduce) are specially developed to process and utilize data in large volumes (thousands of terabytes) and at high velocities (several terabytes per day).
Smart devices and additional instrumentation are adding to the already large volumes of data gathered by the CPI. This data has grown in volume, velocity (speed of accumulation), variety (structured, semi-structured, non-structured), and veracity (spectrum of quality, certainty, and correctness).
Additionally, companies now continuously gather and archive big data about their customers, suppliers, competitors, and relevant ecosystems. This has triggered a seemingly unending wave of platforms, tools, and solutions that promise insights from companies’ big data. Now, the term big data refers not only to the data itself, but also to the associated data-processing solutions, including data extraction, data storage, data cleaning, data mining, data analysis, data visualization, and machine learning.
Although this trend itself is not a bad thing, it has created expectations of big data as a solution to all problems. However, it is not all hype. Big data tools can be used effectively to detect anomalies, diagnose faults, and perform predictive and preventive maintenance, as well as to determine customer preferences, predict market forecasts, and analyze supply chain risks.
Big data is not suitable for developing models of chemical processes, such as distillation, reactors, and heat exchangers, for which rigorous first-principles models either already exist or can be easily developed. Big data models are based on correlation rather than causation, so they cannot be extrapolated outside the range of the data. Moreover, it is difficult to separate data error, uncertainty, and measurement noise from actual phenomena. Plant data can be and has been used to tune first-principles models. A properly tuned first-principles (causation) model is always better than a correlation model developed from big data analytics.
Benefits for the CPI
Smart manufacturing will expand digitalization beyond plant automation to plant operation, R&D, and even nontechnical functions.
Benefits in plant operation include:
- lower operating costs
- reliable availability of assets via early fault detection based on predictive analytics
- lower maintenance costs as preventive maintenance is replaced with predictive maintenance
- optimized supply chains
- less inventory by implementing production planning solutions
- enhanced reliability via early detection of upsets and faults
- less waste
- on-spec quality using APC.
Benefits in R&D include:
- accelerated lab experiments
- improved research collaboration and open innovation networks
- enhanced technology and market intelligence
- reduced time to market.
Faster experiments could be a boon to R&D. When developing new technologies, CPI companies must conduct lab experiments to develop and evaluate various catalysts and generate kinetic reaction rate parameters. These experiments are typically conducted on a few single-reactor setups. Many experiments must be run to test applicable ranges of temperature, pressure, and species compositions and to build reliable kinetic models. This can take several months to more than a year to complete. High-throughput experimentation (HTE) systems incorporate robotics to conduct up to 50 parallel experiments. HTE enables several months of work to be accomplished in several weeks.
Benefits in nontechnical areas include:
- improved customer relationships
- enhanced supplier and distributor experience
- improved talent acquisition
- improved business development.
It is important to track customers’ preferences, comments, and purchase patterns to improve customer relationships. A company’s internal customer-relationship management (CRM) data holds limited information. However, the internet hosts many platforms where customers express their view of products directly or indirectly, including social media, review sites, and discussion forums. Big data analytics can extract useful information about customer preferences from these sources, enabling personalized customer interactions and services.
The benefits to plant operations, R&D, and nontechnical areas are clear, and the CPI will need to embrace this new wave of digital technologies as a necessity. Some decision-makers in the CPI view digitalization as nice to have but not necessary. Digitalization can be expensive and time-intensive, but with the proper knowledge, a realistic strategy, and comprehensive planning, cost and time can be minimized.
Reluctance to transition to digital solutions might also be based on past experiences with legacy software platforms that were proprietary and expensive. Modern digitalization solutions are mostly based on open and free platforms. In addition, pay-per-usage services can minimize hardware costs. In most cases, CPI facilities are already collecting and storing the necessary data.
The primary hurdle is to start using this data and connecting entities to form an IIoT. Data can then be transformed into trackable key performance indicators (KPIs) that can inform and support decisions. Connectivity will bring departments closer and facilitate collaboration. AI-based CRM will enhance customer relationships, and increased mobility will enable remote access. This digital transformation will make companies more knowledgeable, agile, and competitive.
Despite the clear advantages, many decision-makers are still doubtful, indifferent, or maybe a little confused. While some remain skeptical, others believe that they have already digitalized their enterprise because they implemented enterprise resource planning (ERP), APC, and other conventional solutions. Others are reticent to share data, even among their own departments and people. In addition, many have genuine concerns about data security and cyberattacks.
Although confusion and concerns abound, many CPI companies have already started their digital journey. Chemical companies are planning to spend 5% of their annual revenue on digitization, and approximately one-third claim they have already achieved a high level of digitization (2).
Achieving smart manufacturing
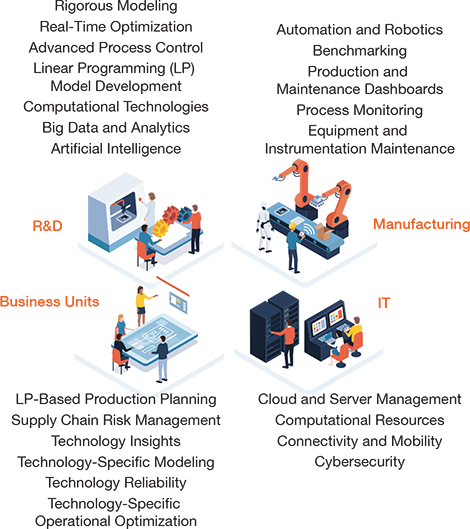
▲Figure 4. Each department has a role to play in a company’s digital journey.
The most expedient and logical way to start a digital journey is to use an industry-specific platform available from a known vendor. Each department should be involved in a unified implementation of digitalization to avoid silos and duplicate work. Each department has a role to play in company-wide digitalization endeavors (Figure 4).
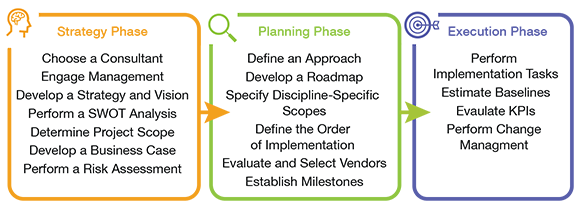
▲Figure 5. Achieving smart manufacturing is a three-phase journey.
To manage this huge undertaking, companies should approach digitalization as a series of phases that include strategy, planning, and execution (Figure 5). A strategy is key to avoid chaos and disorder in the planning and execution phases. A well-formed digitalization strategy establishes limits and ranges for cost, a time period, and the extent and expected outcomes of the project, as well as determines a planning phase team. These boundaries will be determined from a detailed strengths, weaknesses, opportunities, and threats (SWOT) analysis and a high-level quantitative analysis.
The strategy phase should be led by the corporate strategy department or an equivalent corporate-level department, and may benefit from the services of a relevant consultant company. This phase may take two to six months, depending on the size, commitment, and efficiency of the company.
During the planning phase, each department should separately develop a detailed business case or benefits study. These studies can be used to inform priorities, scope, and budget for target departments. At this point, the planning team needs to develop digitalization roadmaps that include approximate timelines for individual departments and the overall enterprise. Consultants can be helpful in determining preliminary milestones for each roadmap, as well as selecting systems, services, and solution providers. They can also assist in setting high-level success criteria for the projects that are planned for the subsequent execution phase.
A detailed plan makes the execution phase much easier. During the execution phase, multiple department-specific projects will be simultaneously implemented. The projects will mostly be executed by the digital solutions providers in coordination with company project teams.
Standard best practices for project management need to be adopted. As digital solutions will most likely change various work processes, the change management may be quite challenging. Therefore, it is crucial to educate relevant staff about the impact of digitalization from the beginning of project execution.
Looking forward
It is important to have a balanced view of smart manufacturing and digitalization. Do not consider it magic or simply all hype. Like any other tools, the benefits of digital solutions depend on how they are selected, implemented, and utilized.
Because digitalization is applicable to many areas of an enterprise, multiple departments may independently initiate their own digital projects. If various departments in your organization have already started digital initiatives, you may want to ensure they are coordinated under a company-wide strategy. Change management is also critical. Finally, remember that many digital solutions extensively utilize technical, commercial, and financial data that requires a good data-stewardship program with dedicated data engineers.
Literature Cited
- Deloitte Consulting LLP, “Digital Transformation: Are Chemical Enterprises Ready?,” www2.deloitte.com/global/en/pages/consumer-industrial-products/articles/digital-transformation-chemical-enterprises-prepare.html (Jan. 2017).
- Sarathy, V., et al., “2017 Chemical Trends,” Strategy&, PwC, London, U.K., www.strategyand.pwc.com/trend/2017-chemicals-industry-trends (2017).
Copyright Permissions
Would you like to reuse content from CEP Magazine? It’s easy to request permission to reuse content. Simply click here to connect instantly to licensing services, where you can choose from a list of options regarding how you would like to reuse the desired content and complete the transaction.