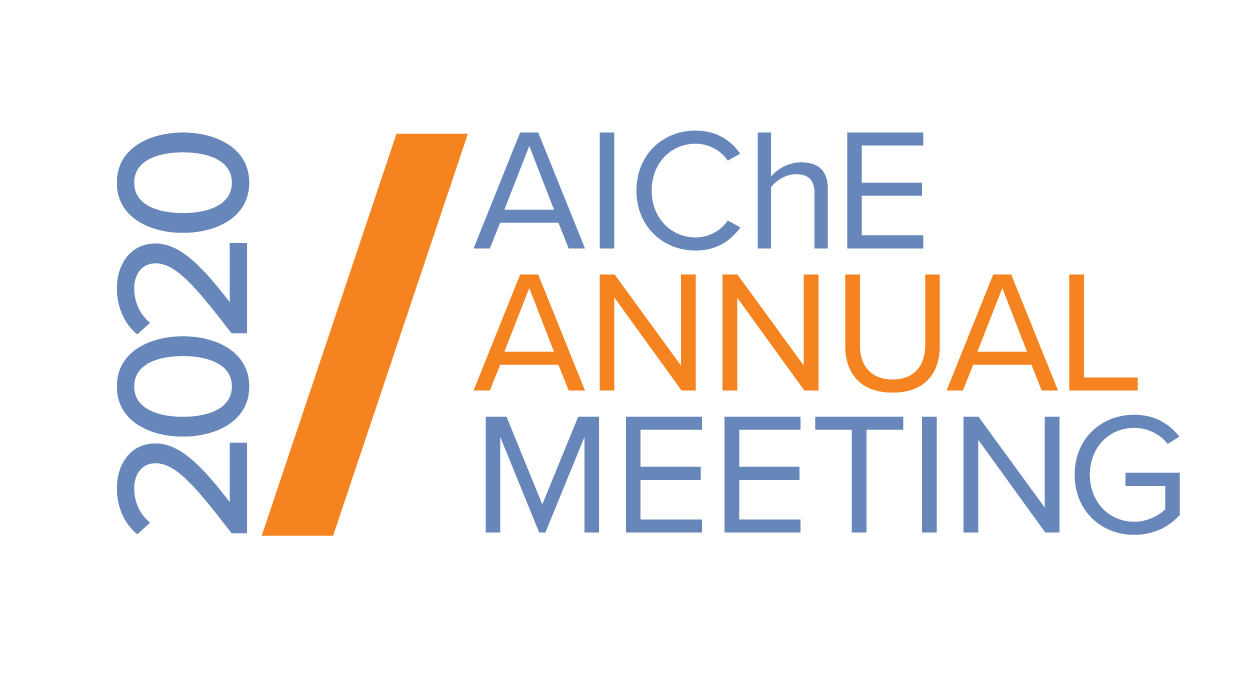
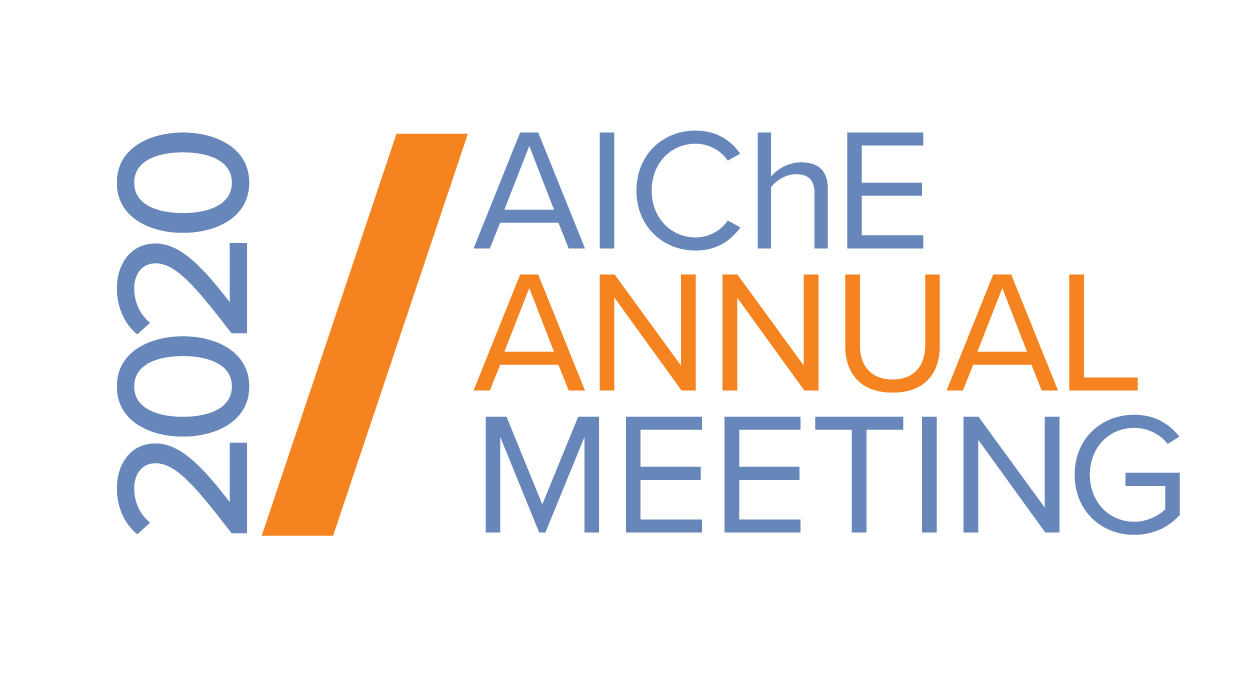
Conversion of biomass feedstocks to hydrocarbon fuels is often fraught with catalyst deactivation due to deposition of coke on catalyst surface or deactivation due to inorganic components of the biomass. Acidic zeolite catalyst get easily deactivated because of the basic oxides in the biomass feedstock. The high aromatic content and macromolecules in the biomass pyrolysis oils also tend to promote carbon formation on the active sites, which eventually lead to their deactivation. We developed a nickel supported on mixed oxide (Ni/RM) catalyst that is more robust than the commercial nickel on silica-alumina catalyst. This new catalyst is regenerable and can be used effectively for hydrodeoxygenation of biomass pyrolysis oils to long chain hydrocarbon fuels. The new catalyst is effective in opening both furan and benzene rings and thus producing hydrocarbon fuels with linear and branched carbon chain length between C6 to C15. The catalyst was not deactivated by inorganic components in the biomass, however, the catalyst activity decreased with deposition of coke on the active sites and required frequent catalyst regeneration through combustion and reduction to restore activity. We developed a machine learning (ML) algorithm which enabled us to reformulate the catalyst and reduce the carbon deposition on the active site. The signature of each nickel supported mixed oxide catalyst (Ni/RM)) was defined as the weight percent of eleven elements (Al, Ca, Fe, K, Mg, Na, Ni, P, S, Si, Ti). These together with the reaction temperature formed the feature vector (input to ML). The output of the ML was coke formation, which was also a performance measure for the designed catalyst. The network relating the ML inputs to the output was either a simple Neural Network or a Convolutional Neural Network (CNN). Using this ML model as a basis, we inverted the model (inverse modeling) to obtain a new Ni/RM catalyst, which was then validated through experiments. The machine learning approached reduced catalyst development time and improved the robustness of the catalyst. Thus, the carbon deposition rate was reduced and catalyst regeneration frequency was also reduced.
Preview Presentation
Presenter(s)
Once the content has been viewed and you have attested to it, you will be able to download and print a certificate for PDH credits.
If you have already viewed this content,
please click here
to login.