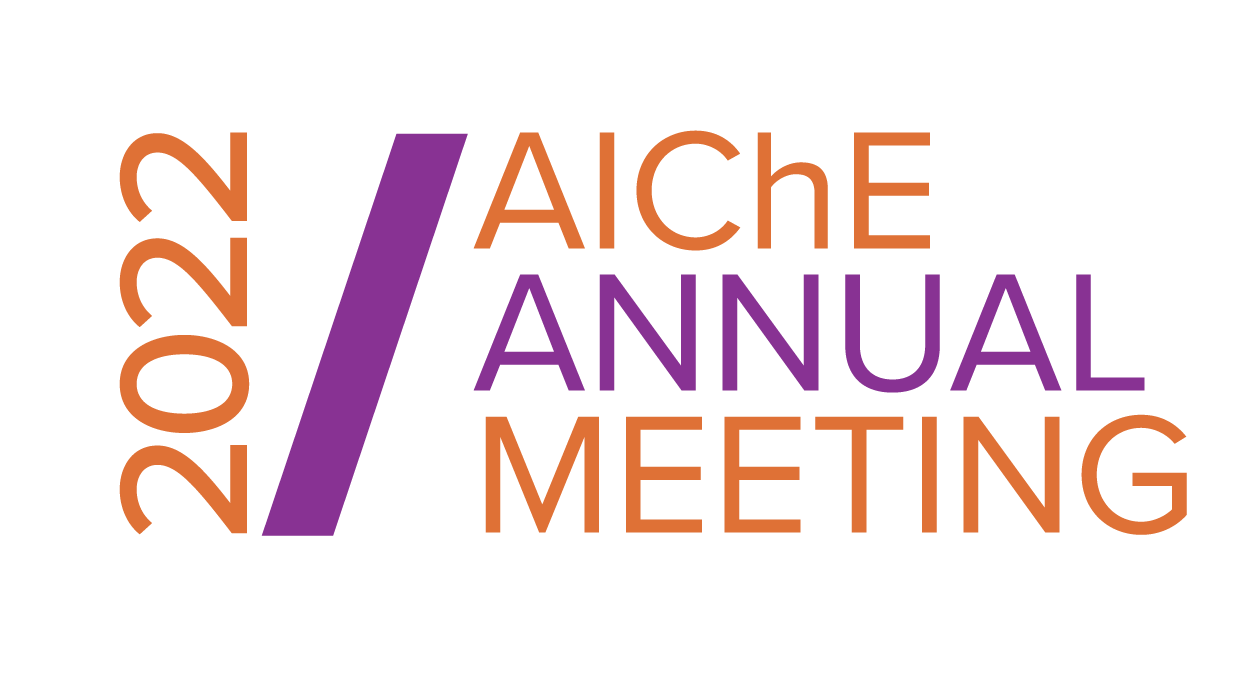
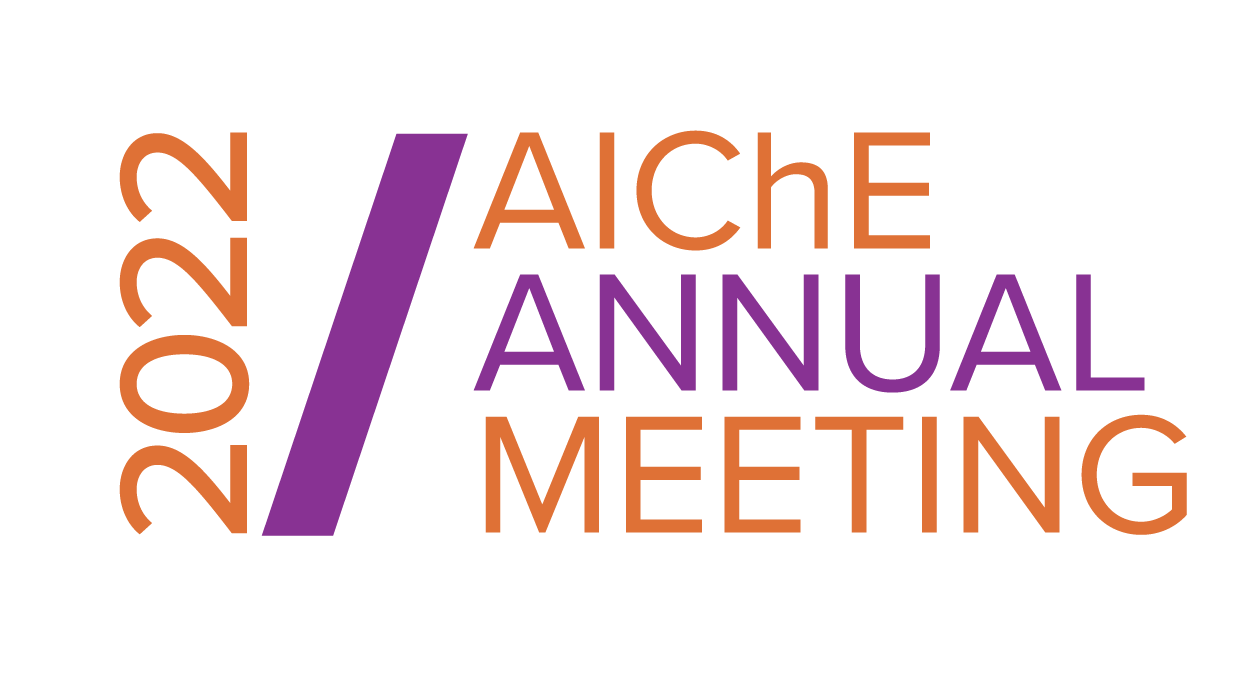
Specific to the pharmaceutical industry, the solubility of active pharmaceutical ingredients (API) in a variety of organic solvents is an important property in the development of new drugs. Many curated databases are available for the aqueous solid solubility of API's as this property is of interest in the initial screening process of potential drugs. Further in the drug development chain during lab-scale synthesis, purification, crystallization, and scale-up from batch to continuous processes, information on the solubility of the API's in organic solvents other than water is required. Despite of the tremendous progress made in machine learning for molecular and material science, for the direct prediction of solid solubility in organic solvents at different temperatures, the application of data driven methods is limited by data abundance. For this reason, data-driven machine learning methods are combined with computational chemistry and thermodynamics for the accurate prediction of solid solubility.
The method uses machine learning for the fast prediction of important properties and thermodynamics to relate those properties to the solid solubility. The machine learning models are used to predict solvation free energy1, solvation enthalpy, Abraham solute parameters2, and aqueous solid solubility at 298K. A transfer learning method combines quantum chemistry and experimental data for the prediction of solvation free energy and enthalpy to broaden the application range of the models to solutes with a higher molar mass1. The Abraham solute parameters are used to calculate the sublimation enthalpy, and the gas and solid phase heat capacities at 298K with correlations recently published by Abraham and Acree3, 4. For temperatures up to 350K, no empirical data is required for the prediction of solid solubility limits. At higher temperatures, the temperature dependence of the dissolution enthalpy has to be accounted for through the temperature dependence of the solvation free energy. To calculate the solvation free energy at higher temperatures we rely on our previously published method5, for which the solvent's critical properties are required.
To train the machine learning submodels and to assess the accuracy and robustness of the new method, several quantum chemical and experimental datasets were constructed or compiled in this work. They are all collected in the SolProp data collection, which is made publicly available on Zenodo (https://zenodo.org/record/5970538). In the new CombiSolu-Exp and CombiSolu-HighT-Exp databases, experimental solid solubility data in pure organic solvents at different temperatures are collected from over 170 different literature sources. They are used to validate the new method for predicting solid solubility limits for more than 6000 datapoints including more than 115 solutes, 95 organic solvents, and temperatures up to 593K. Data from different sources are compared to assess the experimental uncertainty in published solid solubility data. For aqueous solid solubility, the mean-absolute-error (MAE) between different data sources goes up to 0.17 log10(mol/L), while for the solid solubility in organic solvents more than 12% of the duplicated data has an absolute deviation higher than 0.2 log10(mol/L). It should be noted that this rather high experimental uncertainty can significantly affect the perceived performance of the new method and machine learning models.
First, the solid solubility at 298K in different organic solvents is predicted by the proposed model. For 1051 datapoints in the CombiSolu-Exp database (98 solutes and 87 solvents), using only molecular identifiers of the solute and solvent, the solid solubility is predicted with a MAE equal to 0.62 log10(mol/L). This method uses machine learning models to predict the aqueous solid solubility, which is used as a reference to relate the solubility in water to the solubility in other solvents through thermodynamic relationships. Using the experimental solid solubility in ethanol as a reference improves the predictions to a MAE of 0.16 log10(mol/L). However, the experimental measurements in ethanol often originate from the same source as the experimental data measured in other solvents for the same solute. As a result, it is expected that some consistent errors specific to the experimental apparatus or procedure are compensated for and the model performance might be overestimated. Alternatively, we used the experimental aqueous solid solubility from different sources as a reference which led to a MAE of 0.35 log10(mol/L) for the prediction of solid solubility in different organic solvents at 298K.
To relate the solid solubility at 298K with the solid solubility at different temperatures, the model uses the dissolution enthalpy. This value is calculated through thermodynamic relations with the solvation enthalpy and sublimation enthalpy at 298K. Up to 350K, the temperature dependence of the dissolution enthalpy can be neglected and the solvation enthalpy at 298K, predicted by a transfer learning model, can be used for its calculation. The 4922 solid solubility datapoints in the CombiSolu-Exp database (115 solutes and 95 solvents between 243 and 364K) are predicted using only molecular identifiers of the solute and solvent with a MAE equal to 0.99 log10(mol/L). Using experimental solid solubility measured in ethanol as a reference - if available - improves the MAE to 0.29 log10(mol/L). At higher temperatures (>350K), the temperature dependence of the dissolution enthalpy has to be accounted for. This value can be calculated using the temperature dependent solvation enthalpy and the sublimation enthalpy at 298K. The temperature dependent solvation enthalpy can be calculated with our previously published method5 and the solvent’s critical properties. This method is validated with experimental solid solubility data from the CombiSolu-HighT-Exp database for 1306 datapoints, including 67 solutes and 15 solvents. The solubility trend as a function of temperature is captured well for temperatures up to 593K.
The developed model has an excellent performance in predicting solubility trends in different solvents at 298K and as a function of temperature. The performance can be seen in the attached figure for different solutes. Even though the absolute solid solubility prediction can be off in some cases, the ability to predict solubility trends has tremendous applications. With additional user information on the solubility of the solute in one organic solvent at room temperature (298K), the accuracy of the method can be improved. The newly developed method can be accessed through our conda package (https://anaconda.org/fhvermei/solprop_ml) and through our user-friendly web interface (https://rmg.mit.edu/database/solvation/searchSolubility/).
[1] Vermeire, F. H.; Green, W. H., Transfer learning for solvation free energies: From quantum chemistry to experiments. Chemical Engineering Journal 2021, 418, 129307.
[2] Chung, Y.; Vermeire, F. H., et al., Group Contribution and Machine Learning Approaches to Predict Abraham Solute Parameters, Solvation Free Energy, and Solvation Enthalpy. Journal of Chemical Information and Modeling 2022, 62, (3), 433-446.
[3] Abraham, M. H.; Acree, W. E., Estimation of heat capacities of gases, liquids and solids, and heat capacities of vaporization and of sublimation of organic chemicals at 298.15 K. Journal of Molecular Liquids 2020, 317, 113969.
[4] Abraham, M. H.; Acree, W. E., Estimation of enthalpies of sublimation of organic, organometallic and inorganic compounds. Fluid Phase Equilibria 2020, 515, 112575.
[5] Chung, Y.; Gillis, R. J., et al., Temperature-dependent vapor–liquid equilibria and solvation free energy estimation from minimal data. AIChE Journal 2020, 66, (6), e16976.