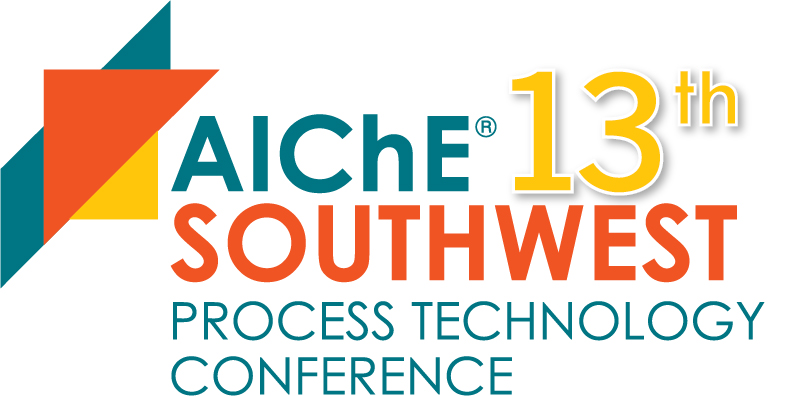
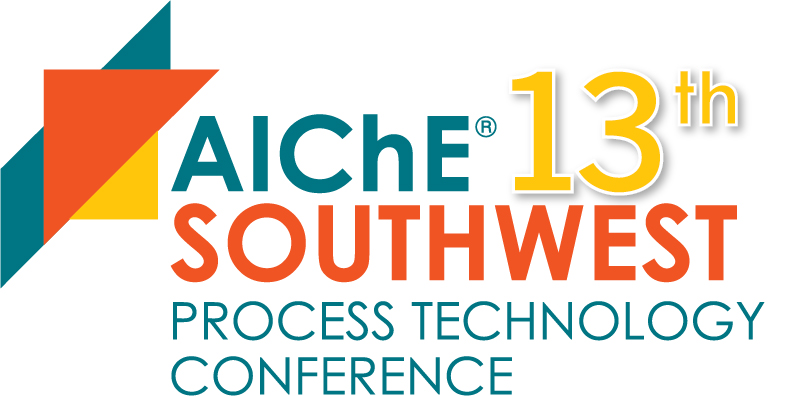
Fault prediction is identified as one of the promising process monitoring tools that predicts the happening of an abnormal event prior to its actual occurrence. Following the prediction of an upcoming fault, a corrective action is taken in advance to minimize the impact of a fault on the process. Consequently, fault prediction schemes can effectively manage faults as compared to fault detection approaches. In this context, we propose a fault prediction method that employs an adaptive model within a dynamic risk assessment framework. Specifically, the proposed method uses operable adaptive sparse identification of systems (OASIS) to model the process and forecast the future dynamics based on the current operating conditions. The OASIS algorithm combines a sparse modeling technique with a deep neural network to adaptively cope with abrupt system changes and predict well even under varying operating conditions. At every sampling time, OASIS forecasts the future process behavior for an n-time step prediction horizon. Utilizing the OASIS prediction, the operational risk is assessed by calculating the probability and severity of a fault that explains the likelihood and the impact of a fault, respectively. Further, the maximum risk value in the considered prediction horizon is monitored for fault prediction. If the maximum risk value exceeds a pre-specified risk threshold, an abnormal event is expected to occur. The proposed method is capable of providing an early fault prediction because of the high prediction accuracy of OASIS. We applied the proposed method to two types of chemical reactors. First, we demonstrated the implementation of the proposed method on a continuous stirred tank reactor (CSTR). Next, we tested the performance of the proposed method through a polyethylene reactor case study.
Preview Presentation
Presenter(s)
Once the content has been viewed and you have attested to it, you will be able to download and print a certificate for PDH credits.
If you have already viewed this content,
please click here
to login.