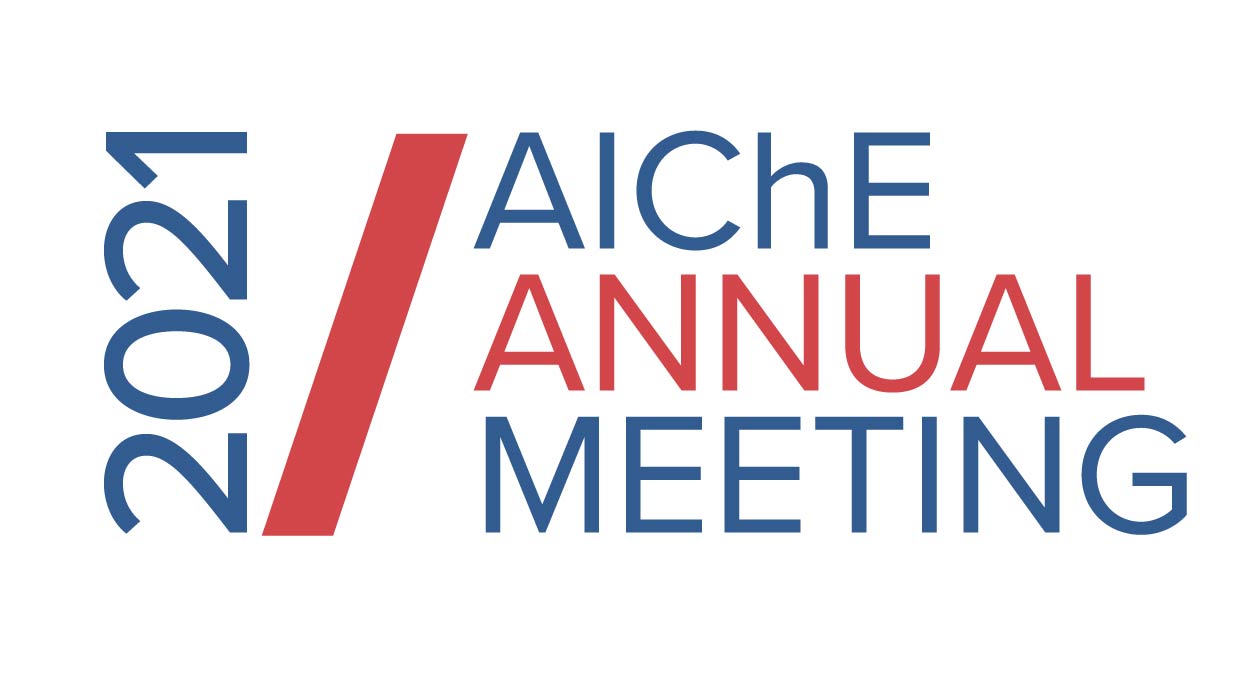
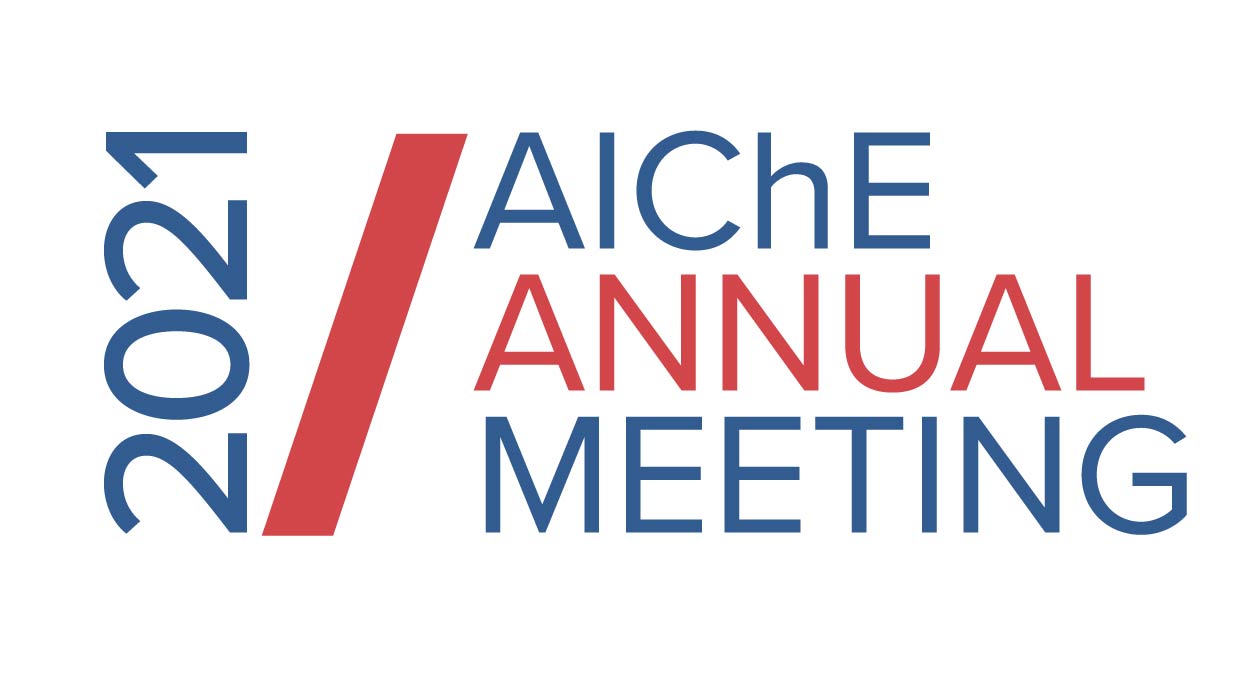
In situ imaging is commonly used to monitor different crystallization processes, characterize the size and shape of product, and detect the presence of undesired shapes or polymorphs [1,2]. There are a series of challenges that hinder the automation of analyzing the captured frames, which is necessary for long-term operation, such as in continuous processes. One challenge is imaging itself; optically dense slurries do not lend themselves to in situ microscopy. Often, ex situ sample loops are employed, which utilize hydrodynamic focusing, backlighting, and dilution to obtain images of in-focus, clearly delineated, non-overlapping crystals [2]. In these cases image quality may be gained at the cost of not fully representing the population and conditions in the sample loop may not match those in the crystallizer. Besides practical problems associated with having a camera inside or in a loop parallel to a vessel, a range of challenges related to the analysis of captured images may prevent gaining usable information from the images. Some of the common challenges in image analysis are illumination, rotation, deformation, overlap, and background clutter.
In this work, we develop a computer vision model based on convolutional neural networks (CNN) that can be combined with high-throughput in situ imaging for monitoring a crystallization process. We are interested in characterizing the state of a system with optically dense slurries where in situ images contain highly over-lapped objects and are hard to analyze with traditional approaches. Our goal is to develop a tool for automated process monitoring that can (1) use in situ images to monitor the state of the process, (2) detect the presence of undesired particles in the slurry, and (3) estimate the location of the undesired particle for further inspection and taking proper control actions. We focus on the application of such a tool in continuous pharmaceuticals manufacturing, where robust monitoring is essential for the production of a pure and high-quality product. We take the production of cephalexin monohydrate (a beta-lactam antibiotic with a large annual production rate of more than 5,000 tons per year [3]) as an example where a byproduct (phenylglcyine) of the reaction may crystallize and contaminate the product in the event of process deviations from the setpoint [4].
Using more than 8000 images, we re-trained the ResNet-18 CNN for the classification of two classes, cephalexin and phenylglcyine particles (the former are needle-like but latter more spherical blobs) with more than 98% accuracy on the validation data [5]. This network is then used to inspect different regions of each image captured from the crystallization vessel using a sliding window approach to detect the presence of phenylglcyine particles. Our analysis of dozens of images shows that the developed model can detect the presence and location of the undesired particles with high accuracy (Fig. 1). Besides discussing our results for this case and a second CNN (ResNet-50), we compare the performance of both models in a range of solid concentrations. We also describe a straightforward procedure for extending this methodology to other crystallization systems using open-source deep learning development frameworks such as TensorFlow.
Fig. 1 The overall process of detecting a PG crystal: A sliding window scans the image, cropping the proposed region in the raw image, passing it through the CNN, generation of feature maps, and classification (top). Results of applying the image analysis model (with ResNet-50 CNN) to a series of in situ images acquired from a crystal slurry (bottom). Solid red boxes correspond to regions that are decided to have a PG particle with 0.8 classification cutoff.
Cited literature
[1] Simon, Levente L., Hajnalka Pataki, GyoÌrgy Marosi, Fabian Meemken, Konrad HungerbuÌhler, Alfons Baiker, Srinivas Tummala et al. "Assessment of recent process analytical technology (PAT) trends: a multiauthor review." Organic Process Research & Development 19, no. 1 (2015): 3-62.
[2] Liu, Tao, Yan Huo, Cai Y. Ma, and Xue Z. Wang. "Sparsity-based image monitoring of crystal size distribution during crystallization." Journal of Crystal Growth 469 (2017): 160-167.
[3] Wegman, Margreth A., Michiel HA Janssen, Fred van Rantwijk, and Roger A. Sheldon. "Towards biocatalytic synthesis of βâlactam antibiotics." Advanced Synthesis & Catalysis 343, no. 6â7 (2001): 559-576.
[4] Salami, Hossein, Colton E. Lagerman, Patrick R. Harris, Matthew A. McDonald, Andreas S. Bommarius, Ronald W. Rousseau, and Martha A. Grover. "Model development for enzymatic reactive crystallization of β-lactam antibiotics: a reactionâdiffusion-crystallization approach." Reaction Chemistry & Engineering 5, no. 11 (2020): 2064-2080.
[5] Salami, Hossein, , Matthew A. McDonald, Andreas S. Bommarius, Ronald W. Rousseau, and Martha A. Grover. "In situ imaging combined with deep learning for crystallization process monitoring: Application to cephalexin production." Organic Process Research and Development (submitted)