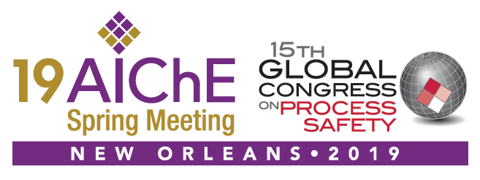
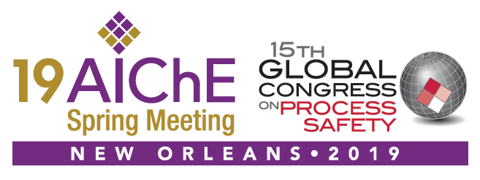
Monitoring equipment deterioration can be a challenge as these parameters cannot be directly measured. Fortunately, state estimation methods are available, which can be utilized in order to estimate the values of these process parameters, and additionally other state variables, including those that cannot be easily measured using sensors. The family of Kalman filter estimation methods, including the Extended Kalman Filters (EKF) and Unscented Kalman Filters (UKF), have been utilized by many in order to carry out effective state and parameter estimation for different applications [2]. Many industrial processes, and pieces of equipment are known to follow nonlinear models. Recently, state estimation through particle filters has also received a reasonable amount of attention.
This work involves monitoring of a process using state estimation methods in order to identify the economic impact of equipment deterioration over time. Application of the algorithm will be demonstrated through a nonlinear example, e.g., a heat exchanger that experiences increasing levels of fouling over time. The presence of fouling causes the overall heat transfer coefficient to decrease, making the heat exchanger less efficient. This in turn forces the heat exchanger to utilize more cooling utility in order to maintain the same set points, thereby increasing operational costs. The example will estimate the parameter, i.e., overall heat transfer coefficient, and the states using different state estimation methods [3]. Additionally, dynamic data will be obtained by simulating the nonlinear heat exchanger in ASPEN Plus Dynamics, in order to enhance of the validity of the proposed algorithm.
References
[1] M.Z. Sheriff, F. Harrou, M. Nounou, Univariate process monitoring using multiscale Shewhart charts, in: 2014 Int. Conf. Control. Decis. Inf. Technol., IEEE, Metz, France, 2014: pp. 435â440.
[2] M.M. Mansouri, H.N. Nounou, M.N. Nounou, Nonlinear control and estimation in induction machine using state estimation techniques, Syst. Sci. Control Eng. 2 (2014) 642â654. doi:http://dx.doi.org/10.1080/21642583.2014.956842.
[3] M.S. Peters, K.D. Timmerhaus, Plant design and economics for chemical engineers, 4th ed., McGraw-Hill, 1991.
Preview Presentation
Presenter(s)
Language
Pricing
Individuals
AIChE Member Credits | 0.5 |
AIChE Pro Members | $19.00 |
Fuels and Petrochemicals Division Members | Free |
AIChE Graduate Student Members | Free |
AIChE Undergraduate Student Members | Free |
AIChE Explorer Members | $29.00 |
Non-Members | $29.00 |