2022 Annual Meeting
(416d) Automatic Strain Sensor Design Via Active Learning and Data Argumentation for Soft Machines
Machine learning (ML) is a versatile tool that builds up a model to make predictions or recommendations, which has been particularly appropriate for the data-rich systems to uncover underlying trends and investigate complex correlations. The exploitation of machine intelligence to research in materials science has gained many interests in the subjects of organic/inorganic catalyst design, drug discovery, and quantum dot synthesis, where a large number of data points can be supplied either computationally or analytically. In contrast, at the device level, the data acquisition rate is primarily limited by the scarcity of high-quality data. For example, substantial challenges exist in developing a prediction model with high accuracy to design piezoresistive strain sensors. First, there are no standardized protocols for the fabrication/characterization of soft strain sensors, and different research laboratories come up with their own fabrication schemes. Thus, the sensor data collected from the literature are with poor consistency and may contain bias. Second, most of recent reports focused on the fabrication of strain sensors enabling real-time monitoring of in-plane strains between 30% to 200%. Such unbalanced data is likely to produce a ML model with restricted prediction capabilities within specific strain ranges, which would not benefit the strain sensor design for many soft machines at extreme strain cases (e.g., < 5% or > 300%). Last, the fabrication of soft strain sensors is normally tedious and time-consuming, so the data acquisition rate is low, making the ML model fall short of data points and present insufficient prediction accuracy.
As a result, it is of high value to address these challenges through a hybrid approach (experimentally and computationally) to construct a ML model at device level, which can realize the concept of automatic strain sensor design for diverse soft machines. In this presentation, a hybrid approach through wet-lab experiments and machine intelligence was developed to automate the design of strain sensors across a wide strain range (from <0.5% to 350%) by developing a high-accuracy prediction model. A three-stage ML framework, including boundary definition, active learning, and data augmentation, was realized to cultivate the prediction ML model with high accuracy. To ensure data quality and balance, 351 delamination tests and 125 nanomaterials-based strain sensors were conducted/fabricated by one lab under identical protocols. After data augmentation and genetic algorithm-based selection, the multi-dimensional dataset of ML-enabled prediction model contained >10,000 real and virtual data points. An ultimate prediction model was finally constructed and able to (1) predict sensor characteristics based on fabrication recipes and (2) recommend feasible fabrication recipes for adequate strain sensors. As final demonstrations, model-suggested strain sensors were integrated into soft gripper and batoid-like swimmer to endow them with real-time sensing capabilities.
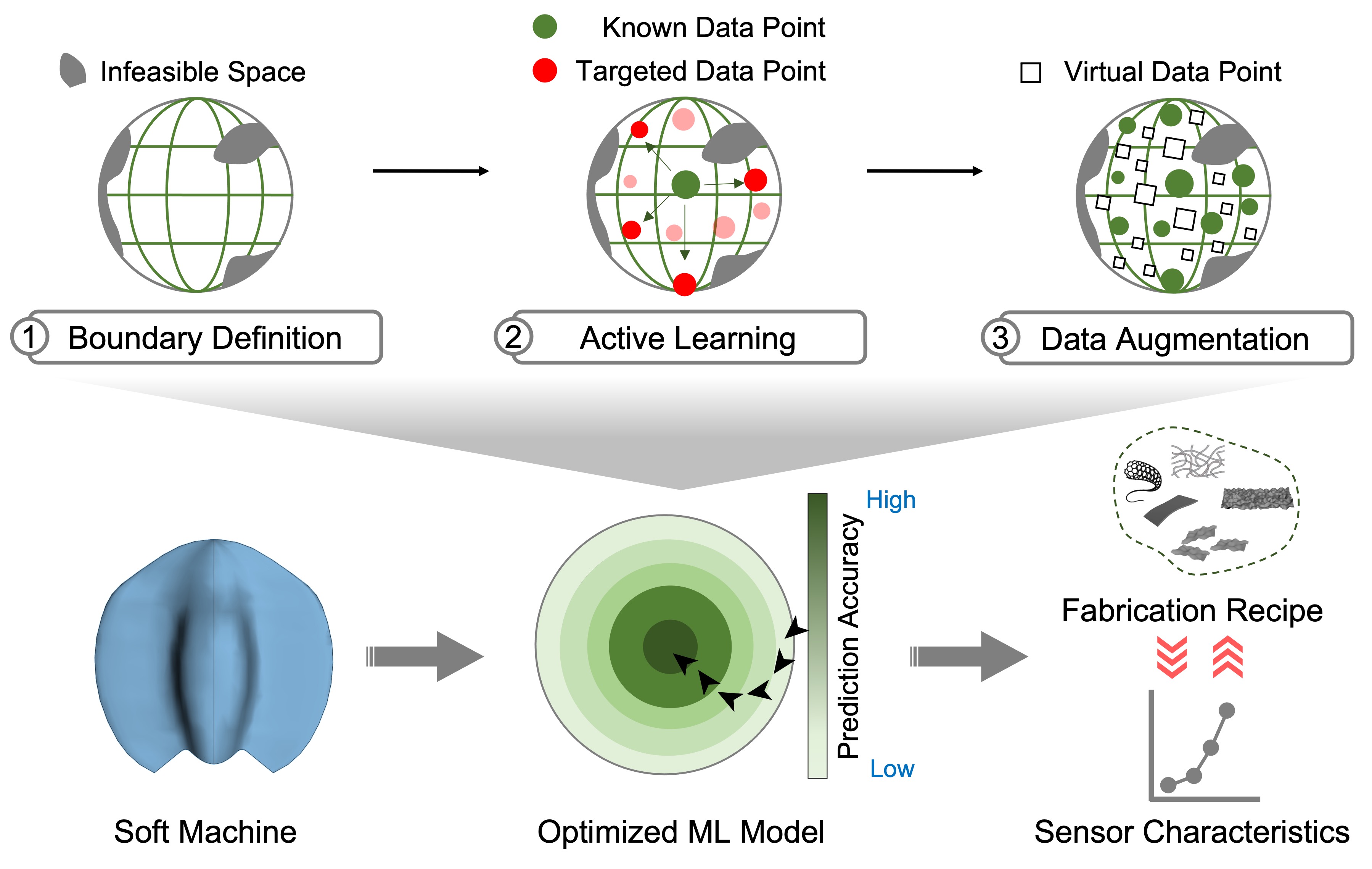
As a result, it is of high value to address these challenges through a hybrid approach (experimentally and computationally) to construct a ML model at device level, which can realize the concept of automatic strain sensor design for diverse soft machines. In this presentation, a hybrid approach through wet-lab experiments and machine intelligence was developed to automate the design of strain sensors across a wide strain range (from <0.5% to 350%) by developing a high-accuracy prediction model. A three-stage ML framework, including boundary definition, active learning, and data augmentation, was realized to cultivate the prediction ML model with high accuracy. To ensure data quality and balance, 351 delamination tests and 125 nanomaterials-based strain sensors were conducted/fabricated by one lab under identical protocols. After data augmentation and genetic algorithm-based selection, the multi-dimensional dataset of ML-enabled prediction model contained >10,000 real and virtual data points. An ultimate prediction model was finally constructed and able to (1) predict sensor characteristics based on fabrication recipes and (2) recommend feasible fabrication recipes for adequate strain sensors. As final demonstrations, model-suggested strain sensors were integrated into soft gripper and batoid-like swimmer to endow them with real-time sensing capabilities.
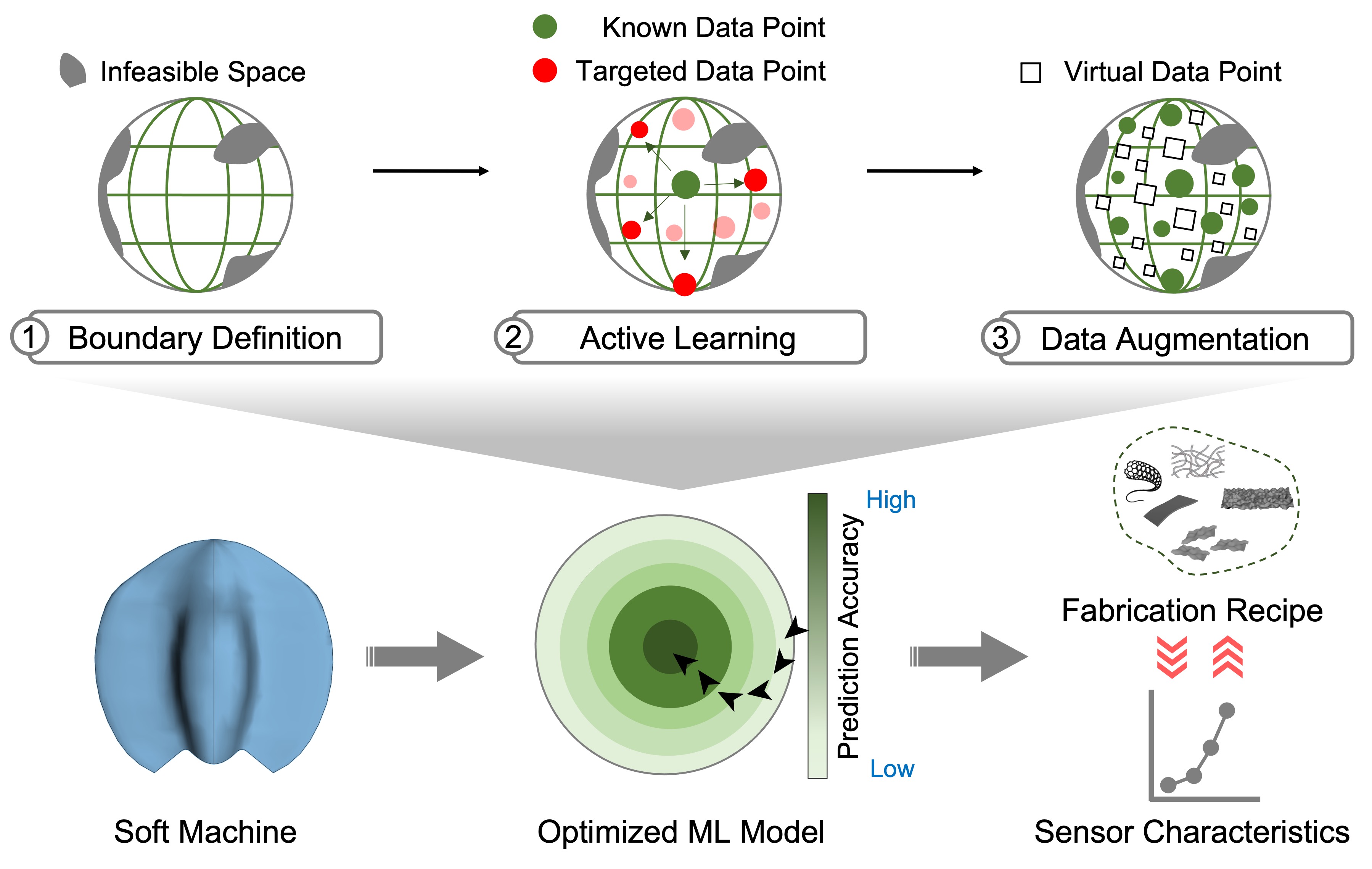